Compressed-sensing magnetic resonance imaging reconstruction method for correcting regularization parameters
A magnetic resonance imaging and compressed sensing technology, applied in the field of image processing, can solve the problems of high operating costs, reduced clinical throughput, reduced scanning time and reconstruction time, and reduced equipment utilization, so as to reduce the number of samples and reduce the cost of magnetic resonance imaging. time, reducing the effect of data transmission
- Summary
- Abstract
- Description
- Claims
- Application Information
AI Technical Summary
Problems solved by technology
Method used
Image
Examples
specific Embodiment approach 1
[0046] A compressed sensing MRI reconstruction method with modified regularization parameters, such as figure 1 shown, including the following steps:
[0047] Step a, obtaining part of K-space data;
[0048] Step b, using the total variational transformation model theory to construct an objective function for magnetic resonance image reconstruction;
[0049] Step c, according to the constructed objective function, using the solution method of the alternating direction multiplier algorithm, introducing an auxiliary variable regularization coefficient to balance the regular term and the data constraint term, and transforming the optimization problem of the objective function into the solution problem of the sub-function;
[0050] Step d, update the alternate direction multiplier algorithm subproblems;
[0051] Step e, update the Lagrange multiplier;
[0052] Step f, add correction factor and , modify the regularization parameter The value of , balance the regular item a...
specific Embodiment approach 2
[0103] The embodiment reconstruction algorithm selects the ADMM algorithm, and the reconstructed image selects the brain MRI image, and the image size is , and its implementation steps are as follows:
[0104] 1. The known measurement matrix uses a radial measurement matrix to initialize the under-sampled K-space observation data, perform inverse Fourier transform on it, and obtain the reconstructed initialization image x.
[0105] 2. Initialization parameters , , , , , , , , .
[0106] 3. Use the Alternate Multiplier Algorithm to solve the subproblem and update the initial value of the Lagrange multiplier , :
[0107]
[0108] 4. Use regularization parameters Solve the subproblem:
[0109]
[0110] 5. Add correction factor , modifying the regularization coefficient to solve the subproblem:
[0111]
[0112] 6. Set the dual reconstruction index of peak signal-to-noise ratio and structural similarity, and judge whether to add the correcti...
PUM
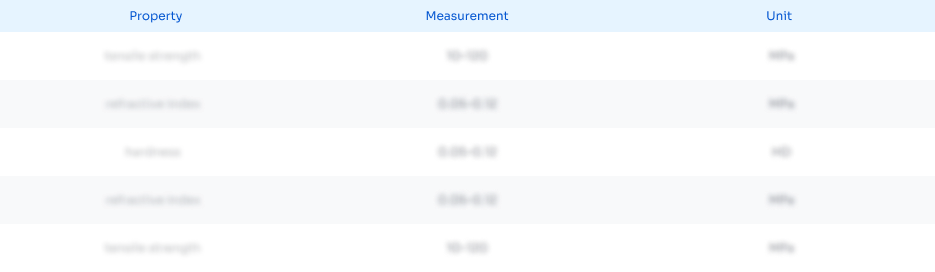
Abstract
Description
Claims
Application Information
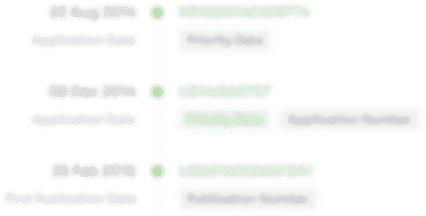
- Generate Ideas
- Intellectual Property
- Life Sciences
- Materials
- Tech Scout
- Unparalleled Data Quality
- Higher Quality Content
- 60% Fewer Hallucinations
Browse by: Latest US Patents, China's latest patents, Technical Efficacy Thesaurus, Application Domain, Technology Topic, Popular Technical Reports.
© 2025 PatSnap. All rights reserved.Legal|Privacy policy|Modern Slavery Act Transparency Statement|Sitemap|About US| Contact US: help@patsnap.com