Fast shift invariant CPD (canonical polyadic decomposition) method applicable to multi-testee fMRI (functional magnetic resonance imaging) data analysis
A multi-subject, shift-invariant technology, applied in the field of medical signal processing, can solve the problems of large data volume, slow running speed, and high memory requirements, achieve good development prospects and improve the effect of separation performance
- Summary
- Abstract
- Description
- Claims
- Application Information
AI Technical Summary
Problems solved by technology
Method used
Image
Examples
Embodiment Construction
[0029] A specific embodiment of the present invention will be described in detail below in conjunction with the technical scheme and accompanying drawings.
[0030] There are currently 10 subjects performing the fMRI data collected under the finger tapping task, that is, K=10. Each subject underwent J=165 scans, and each scan collected 53×63×46 whole-brain data, removed data voxels outside the brain, and kept data voxels I=59610 in the brain. Assuming that the number of components sharing SM and TC components is D = 30, the steps of multi-subject fMRI data analysis using the present invention are shown in the accompanying drawings.
[0031]Step 1: Input multi-subject fMRI data
[0032] The second step: initialization. Randomly initialize shared TC components Shared SM ingredients Subject intensity initial subject delay is a zero matrix, the number of iterations iter=0, the relative error Δε iter = 1, calculate the iteration error ε according to formula (1) iter ....
PUM
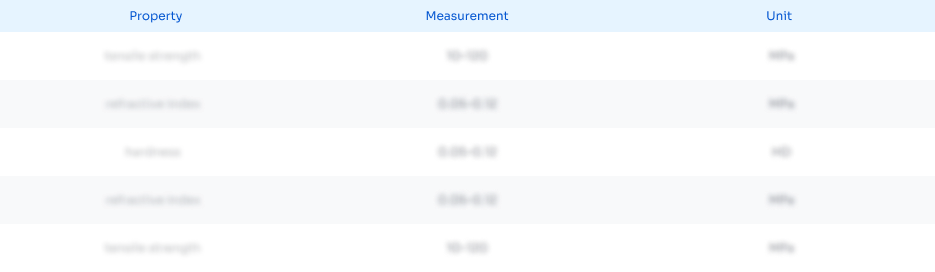
Abstract
Description
Claims
Application Information
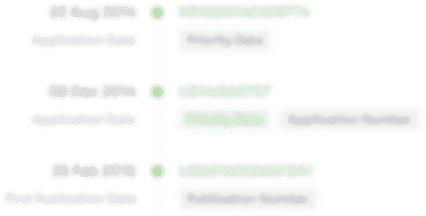
- R&D Engineer
- R&D Manager
- IP Professional
- Industry Leading Data Capabilities
- Powerful AI technology
- Patent DNA Extraction
Browse by: Latest US Patents, China's latest patents, Technical Efficacy Thesaurus, Application Domain, Technology Topic.
© 2024 PatSnap. All rights reserved.Legal|Privacy policy|Modern Slavery Act Transparency Statement|Sitemap