An intelligent medical image segmentation method based on three-dimensional reconstruction
A medical imaging and three-dimensional reconstruction technology, applied in image analysis, image enhancement, image data processing, etc., can solve the problems of not considering image position information, difficult to meet segmentation accuracy, difficult to obtain segmentation results, etc., to reduce the amount of calculation and operation. Time, good segmentation effect, and the effect of ensuring accuracy
- Summary
- Abstract
- Description
- Claims
- Application Information
AI Technical Summary
Problems solved by technology
Method used
Image
Examples
Embodiment Construction
[0059] The present invention will be further described below in conjunction with specific examples.
[0060] Such as figure 1 As shown, the intelligent segmentation method of medical image tissue based on three-dimensional reconstruction provided by the present invention includes the following steps:
[0061] The first step is to input a set of medical image sequences in DICOM format, and perform windowing and Gaussian smoothing on the image sequences in the preprocessing stage. The so-called window adjustment is to convert the gray value in the window in the image data to the value in the brightest and darkest range when displayed according to the predicted window width and window level, and set the part higher than the gray range of the window to the lowest. Bright, and the part below the grayscale range of the window is set to the darkest. The window adjustment formula is as follows:
[0062]
[0063] Among them, F' xyz is the gray value of the pixel at the three-dim...
PUM
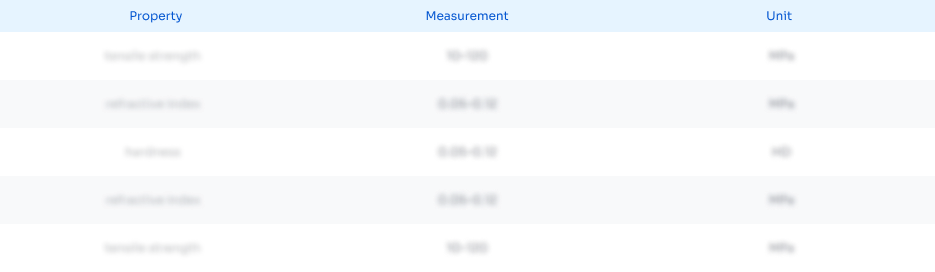
Abstract
Description
Claims
Application Information
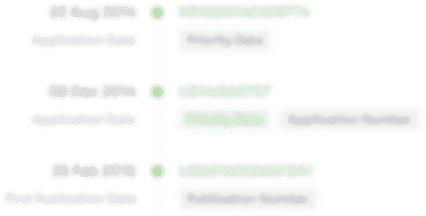
- R&D
- Intellectual Property
- Life Sciences
- Materials
- Tech Scout
- Unparalleled Data Quality
- Higher Quality Content
- 60% Fewer Hallucinations
Browse by: Latest US Patents, China's latest patents, Technical Efficacy Thesaurus, Application Domain, Technology Topic, Popular Technical Reports.
© 2025 PatSnap. All rights reserved.Legal|Privacy policy|Modern Slavery Act Transparency Statement|Sitemap|About US| Contact US: help@patsnap.com