A machine learning-based equipment fault detection method
A technology of equipment failure and detection method, which is applied in the direction of instruments, computer parts, structured data retrieval, etc., can solve the problems of not being widely applicable to data types, low degree of automation, and inability to automatically detect, so as to prevent the occurrence of mechanical failures, The effect of improving economic benefits and improving production efficiency
- Summary
- Abstract
- Description
- Claims
- Application Information
AI Technical Summary
Problems solved by technology
Method used
Image
Examples
Embodiment 1
[0039] Such as figure 1 As shown, the present embodiment provides a machine learning-based device fault detection method, comprising the following steps:
[0040] S1. Acquiring the collected initial data of the faulty equipment;
[0041] S2. Perform a cleaning operation on the initial data, and perform secondary screening on the initial data after the cleaning operation is completed to obtain pre-data;
[0042] S3. Optimizing the pre-data by genetic algorithm, randomly generating multiple starting points from the pre-analyzed data, and then retrieving the starting points at the same time, and outputting the retrieval results;
[0043] S4. Weight the retrieval results by AdaBoost meta-algorithm, and classify the data to obtain the initial result set;
[0044] S5. Classify the initial result set successively according to the set weight of the samples in each classifier, so as to obtain the weighted average result;
[0045] S6. By comparing the weighted average result and the ...
Embodiment 2
[0049] On the basis of Example 1, such as figure 1 As shown, the present embodiment provides a machine learning-based device fault detection method, comprising the following steps:
[0050] S1. Acquiring the collected initial data of the faulty equipment;
[0051] S2. Perform a cleaning operation on the initial data, and perform secondary screening on the initial data after the cleaning operation is completed to obtain pre-data;
[0052]S3. Optimizing the pre-data by genetic algorithm, randomly generating multiple starting points from the pre-analyzed data, and then retrieving the starting points at the same time, and outputting the retrieval results;
[0053] S4. Weight the retrieval results by AdaBoost meta-algorithm, and classify the data to obtain the initial result set;
[0054] S5. Classify the initial result set successively according to the set weight of the samples in each classifier, so as to obtain the weighted average result;
[0055] S6. By comparing the weight...
Embodiment 3
[0060] On the basis of Example 1, such as figure 1 As shown, the present embodiment provides a machine learning-based device fault detection method, comprising the following steps:
[0061] S1. Acquiring the collected initial data of the faulty equipment;
[0062] S2. Perform a cleaning operation on the initial data, and perform secondary screening on the initial data after the cleaning operation is completed to obtain pre-data;
[0063] S3. Optimizing the pre-data by genetic algorithm, randomly generating multiple starting points from the pre-analyzed data, and then retrieving the starting points at the same time, and outputting the retrieval results;
[0064] S4. Weight the retrieval results by AdaBoost meta-algorithm, and classify the data to obtain the initial result set;
[0065] S5. Classify the initial result set successively according to the set weight of the samples in each classifier, so as to obtain the weighted average result;
[0066] S6. By comparing the weigh...
PUM
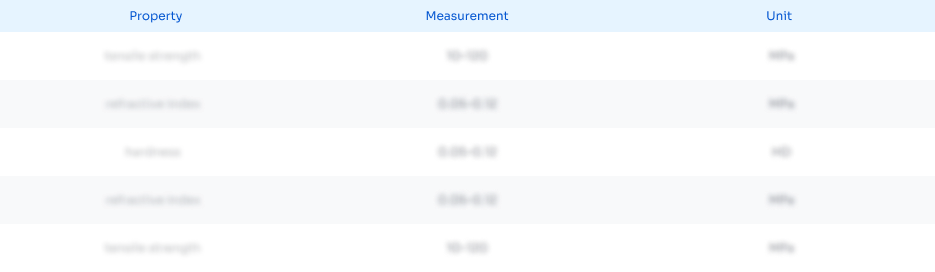
Abstract
Description
Claims
Application Information
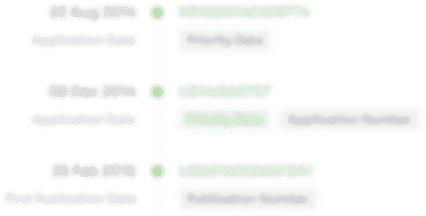
- R&D
- Intellectual Property
- Life Sciences
- Materials
- Tech Scout
- Unparalleled Data Quality
- Higher Quality Content
- 60% Fewer Hallucinations
Browse by: Latest US Patents, China's latest patents, Technical Efficacy Thesaurus, Application Domain, Technology Topic, Popular Technical Reports.
© 2025 PatSnap. All rights reserved.Legal|Privacy policy|Modern Slavery Act Transparency Statement|Sitemap|About US| Contact US: help@patsnap.com