A low-rank sparse optimization target segmentation method under Laplacian regularization constraint
A sparse optimization and target segmentation technology, applied in the field of computer vision, can solve the problems of low target segmentation accuracy, errors, and no consideration of the spatial correlation of calculated elements, etc., to reduce complexity, improve accuracy, and improve robustness Effect
- Summary
- Abstract
- Description
- Claims
- Application Information
AI Technical Summary
Problems solved by technology
Method used
Image
Examples
Embodiment
[0046] Such as Figure 1-Figure 2 As shown, the present invention provides a target segmentation method for low-rank sparse optimization under the Laplace regular constraint, and its specific implementation steps are as follows:
[0047] (S1) Use SLIC algorithm to divide each frame of image in the video into N super pixels;
[0048] (S2) Extract the features of different convolution layers in the VGG network, calculate the average feature of each super pixel as the feature of the super pixel, and set up the image feature matrix X, the establishment method of the image feature matrix X is:
[0049] Calculate the average feature of each superpixel
[0050]
[0051] Create an image feature matrix X:
[0052]
[0053] Among them, f j The feature vector built for the superpixel, is the number of pixels contained in super pixel i, ∑ j f j In order to accumulate the pixel feature vector contained in a certain super pixel, j=1, 2...n 1 ,n 1 is the number of pixels in ...
PUM
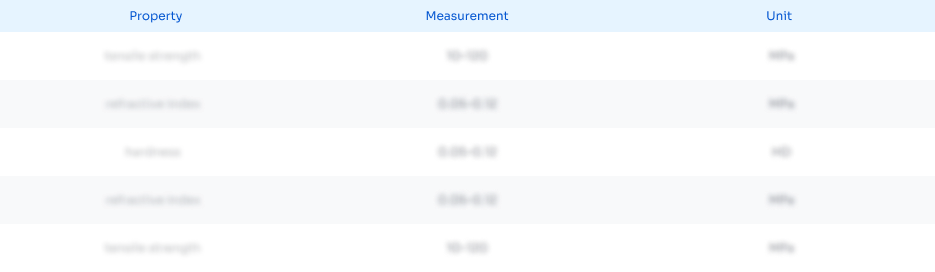
Abstract
Description
Claims
Application Information
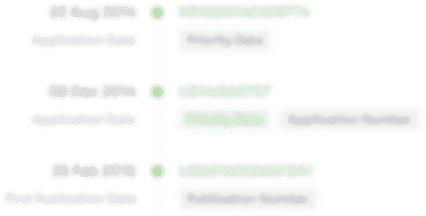
- Generate Ideas
- Intellectual Property
- Life Sciences
- Materials
- Tech Scout
- Unparalleled Data Quality
- Higher Quality Content
- 60% Fewer Hallucinations
Browse by: Latest US Patents, China's latest patents, Technical Efficacy Thesaurus, Application Domain, Technology Topic, Popular Technical Reports.
© 2025 PatSnap. All rights reserved.Legal|Privacy policy|Modern Slavery Act Transparency Statement|Sitemap|About US| Contact US: help@patsnap.com