CT image detection method and device based on deep learning and control equipment
A CT image and deep learning technology, applied in image analysis, image data processing, instruments, etc., can solve the problems that the precision is difficult to reach the expert level, the generalization ability is not strong, and it is difficult to apply clinically, so as to achieve fast response speed and ease Effects of medical pressure and difficulty in procrastinating illness
- Summary
- Abstract
- Description
- Claims
- Application Information
AI Technical Summary
Problems solved by technology
Method used
Image
Examples
Embodiment 1
[0041] This embodiment takes the CT image of liver cancer as an example for illustration, but it does not mean that the present invention can only be applied to the detection of liver cancer. The present invention can be widely used in the detection of CT images of different types of diseases. disease detection probability.
[0042] CT images reflect the human body's absorption coefficient of X-rays, but the density difference of each tissue structure, that is, the relative density, if a tissue has a lesion, its density will change, but because the comparison of the absorption coefficient is very cumbersome, the tissue and organ The absorption coefficient of X-rays is converted into CT value, and the unit is Hu. The CT value of water is 0Hu, and other different tissue densities are compared with it. The density greater than water is defined as a positive value, and the density smaller than water is defined as a negative value.
[0043] like figure 1 As shown, it is a flow cha...
Embodiment 2
[0074] like Figure 8 As shown, it is a structural block diagram of the CT image detection device based on deep learning in this embodiment, including: a preprocessing module: used to preprocess the original image to obtain an image to be detected; an image detection model acquisition module: used to build a neural network detection model, and perform model training to obtain a neural network CT image detection model; output detection result module: used to input the image to be detected into the neural network CT image detection model to obtain detection results.
[0075] On the other hand, the present invention also includes a deep learning-based CT image detection control device, including: at least one processor; and a memory connected to at least one processor; wherein, the memory stores information that can be processed by at least one The instructions executed by the processor are executed by at least one processor, so that the at least one processor can execute the met...
PUM
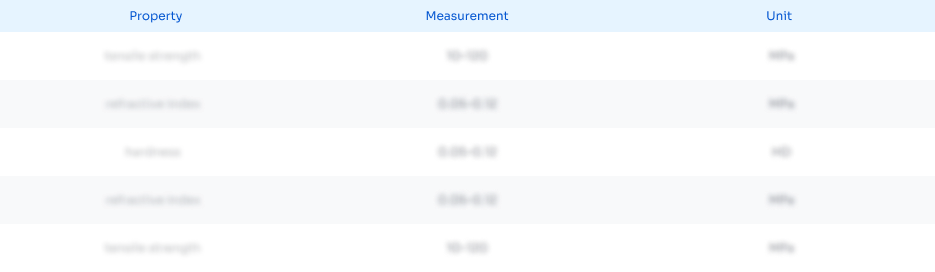
Abstract
Description
Claims
Application Information
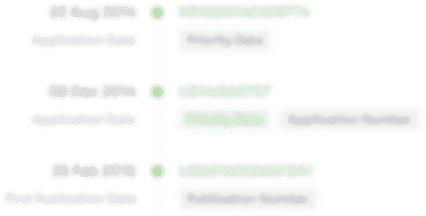
- R&D Engineer
- R&D Manager
- IP Professional
- Industry Leading Data Capabilities
- Powerful AI technology
- Patent DNA Extraction
Browse by: Latest US Patents, China's latest patents, Technical Efficacy Thesaurus, Application Domain, Technology Topic.
© 2024 PatSnap. All rights reserved.Legal|Privacy policy|Modern Slavery Act Transparency Statement|Sitemap