Cyanobacterial bloom prediction method based on recursion time sequence deep belief network
A deep belief network and cyanobacterial bloom technology, which is applied in the field of cyanobacterial bloom prediction based on recursive time series deep belief network, can solve the problems of low accuracy of algal bloom prediction and insufficient number of samples.
- Summary
- Abstract
- Description
- Claims
- Application Information
AI Technical Summary
Problems solved by technology
Method used
Image
Examples
example 1
[0129] Taking the data of algae density, influencing factors total nitrogen, dissolved oxygen and water temperature in the Taihu Lake Basin of Jiangsu Province as an example, the method proposed by the present invention is used to predict cyanobacteria blooms. Taking the observation data of Taihu Lake from June 2009 to November 2010 as an example, after data screening and normalization processing, a total of 5016 samples of algae density data and three samples of influencing factors in 500 days were selected, each of which The influencing factors consisted of 5014 samples and divided them into two groups. The first set of sample data consists of 4008 samples of algae density and data of influencing factors total nitrogen, dissolved oxygen and water temperature, and each influencing factor data is composed of 4007 samples. The second set of sample data consists of 1008 samples of algae density and the data of the influencing factors total nitrogen, dissolved oxygen and water te...
PUM
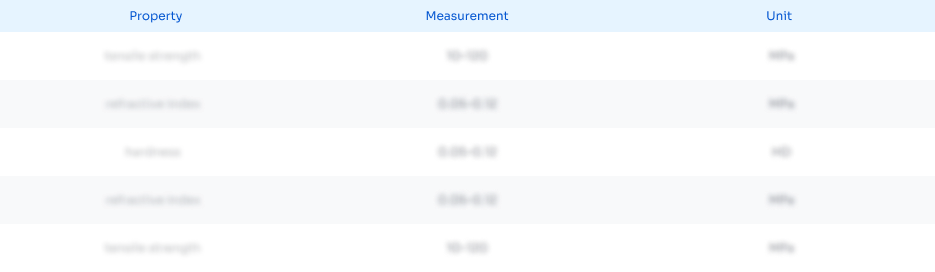
Abstract
Description
Claims
Application Information
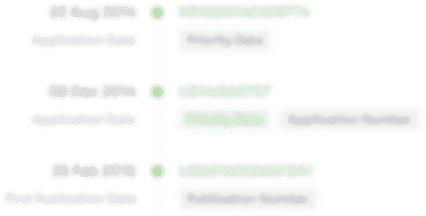
- R&D
- Intellectual Property
- Life Sciences
- Materials
- Tech Scout
- Unparalleled Data Quality
- Higher Quality Content
- 60% Fewer Hallucinations
Browse by: Latest US Patents, China's latest patents, Technical Efficacy Thesaurus, Application Domain, Technology Topic, Popular Technical Reports.
© 2025 PatSnap. All rights reserved.Legal|Privacy policy|Modern Slavery Act Transparency Statement|Sitemap|About US| Contact US: help@patsnap.com