An Image Contour Detection Method Based on Multi-level Feature Channel Optimal Coding
An image contour and channel optimization technology, applied in image analysis, image data processing, instruments, etc., can solve problems such as missing learning process, generalization of feature information, and non-level spiking neural network model.
- Summary
- Abstract
- Description
- Claims
- Application Information
AI Technical Summary
Problems solved by technology
Method used
Image
Examples
Embodiment Construction
[0047] The specific implementation process of the present invention will be described below in conjunction with the accompanying drawings, as figure 1 as shown,
[0048] Step 1: Obtain the primary contour response of the input image I(x,y). First calculate the Gabor filter response of the input image I(x,y), and the result is denoted as As shown in formulas (11) to (14).
[0049]
[0050]
[0051]
[0052]
[0053] In the formula: Represents the Gabor feature information obtained by the image I(x,y) through the Gabor filter on the scale m and direction θ=nπ / K; σ x ,σ y Represent the standard deviation of the Gabor wavelet basis function along the x-axis and y-axis respectively; ω is the complex modulation frequency of the Gaussian function; take ψ(x,y) as the mother wavelet, and obtain the Gabor filter ψ by performing scale and rotation transformation on it m,n (x, y); where u, v are ψ m,n (x, y) template size; m=0,...,S-1, n=0,...,K-1, S and K represent th...
PUM
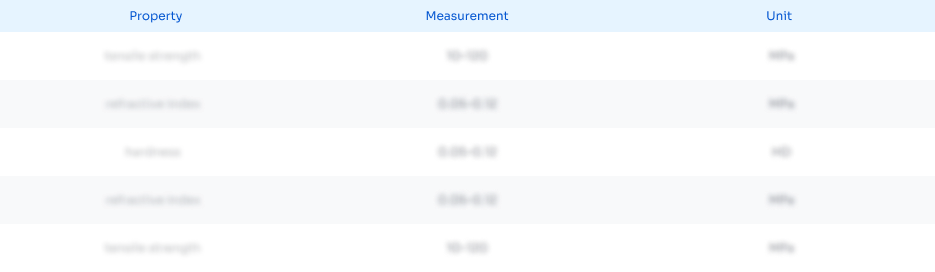
Abstract
Description
Claims
Application Information
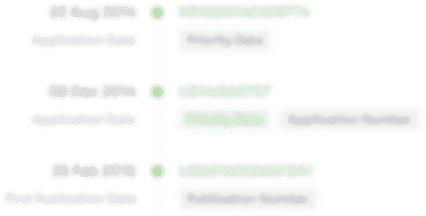
- R&D Engineer
- R&D Manager
- IP Professional
- Industry Leading Data Capabilities
- Powerful AI technology
- Patent DNA Extraction
Browse by: Latest US Patents, China's latest patents, Technical Efficacy Thesaurus, Application Domain, Technology Topic, Popular Technical Reports.
© 2024 PatSnap. All rights reserved.Legal|Privacy policy|Modern Slavery Act Transparency Statement|Sitemap|About US| Contact US: help@patsnap.com