A handwritten picture classification method based on a quantum neural network
A quantum neural and image classification technology, which is applied in neural learning methods, biological neural network models, neural architectures, etc., can solve problems such as large amount of effort, difficult handwritten image classification, difficulty in guaranteeing the reliability and stability of classification results, etc., to achieve guaranteed Reliability and stability, improving recognition speed and recognition accuracy, and reducing difficulty
- Summary
- Abstract
- Description
- Claims
- Application Information
AI Technical Summary
Problems solved by technology
Method used
Image
Examples
Embodiment Construction
[0031] The present invention will be described in further detail below in conjunction with the accompanying drawings.
[0032] Refer to attached figure 1 , further describe in detail the steps realized by the present invention.
[0033] Step 1, extracting handwritten image features.
[0034] Randomly select 60,000 pictures from the handwritten picture database, and the size of each handwritten picture is 28×28 pixels; from each handwritten picture, the pixels of each row are formed into a vector, and all the vectors are formed into the features of the handwritten picture .
[0035] Divide 60,000 handwritten image features into training set and test set, with sizes of 10,000 and 50,000, respectively.
[0036] Step 2, constructing the particle population of the binary quantum particle swarm optimization algorithm.
[0037] Set the number of particles in the binary quantum particle swarm optimization algorithm to 30, and determine the position information of each particle acc...
PUM
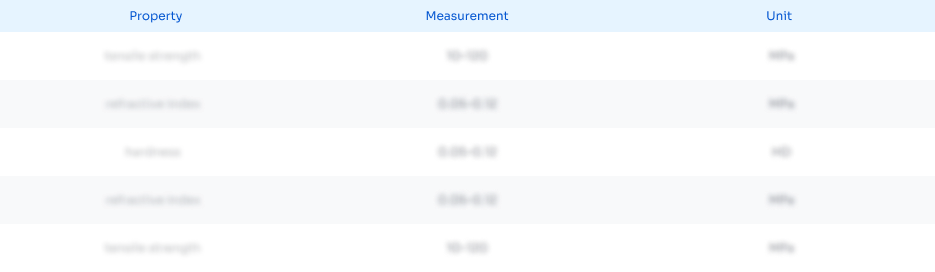
Abstract
Description
Claims
Application Information
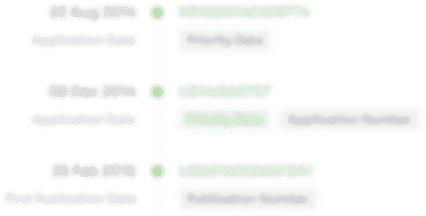
- R&D Engineer
- R&D Manager
- IP Professional
- Industry Leading Data Capabilities
- Powerful AI technology
- Patent DNA Extraction
Browse by: Latest US Patents, China's latest patents, Technical Efficacy Thesaurus, Application Domain, Technology Topic, Popular Technical Reports.
© 2024 PatSnap. All rights reserved.Legal|Privacy policy|Modern Slavery Act Transparency Statement|Sitemap|About US| Contact US: help@patsnap.com