A semi-supervised image instance segmentation method based on stepwise adversarial learning
A semi-supervised, image-based technology, applied in image analysis, image data processing, instruments, etc., can solve technical difficulties and other problems, achieve the effect of reducing workload and improving segmentation performance
- Summary
- Abstract
- Description
- Claims
- Application Information
AI Technical Summary
Problems solved by technology
Method used
Image
Examples
Embodiment Construction
[0035] In order to make the object, technical solution and advantages of the present invention clearer, the specific implementation cases of the present invention will be described below in conjunction with the accompanying drawings.
[0036] The invention discloses a semi-supervised image instance segmentation method based on step-by-step adversarial learning, such as figure 1 shown, including the following steps:
[0037] Step 1. Collect multiple image samples to be segmented, and label some of them to obtain the labeled data set {X l , Y l}, where X l represents a collection of images, Y l Represents a mask label set; unlabeled samples form an unlabeled data set X u ;
[0038] Build an instance segmentation model, the instance segmentation model is Mask R-CNN, its structure is as follows figure 2 As shown, its backbone network is the feature pyramid network FPN; Mask R-CNN also includes the region generation network RPN, RolAlign, and deconvolution network Deconv; th...
PUM
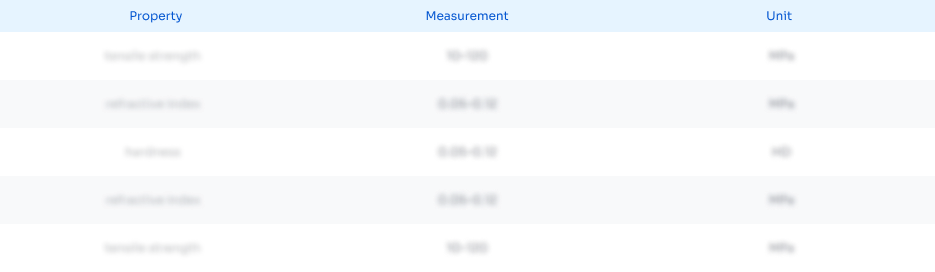
Abstract
Description
Claims
Application Information
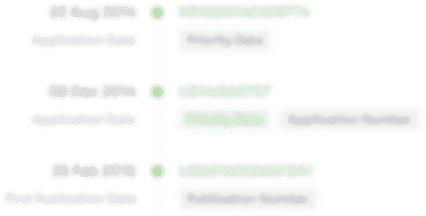
- R&D Engineer
- R&D Manager
- IP Professional
- Industry Leading Data Capabilities
- Powerful AI technology
- Patent DNA Extraction
Browse by: Latest US Patents, China's latest patents, Technical Efficacy Thesaurus, Application Domain, Technology Topic.
© 2024 PatSnap. All rights reserved.Legal|Privacy policy|Modern Slavery Act Transparency Statement|Sitemap