Multi- objective organ-at-risk automatic segmentation method, device and system based on deep learning
A technology of deep learning and automatic segmentation, which is applied in the direction of instruments, image analysis, image data processing, etc., can solve the problems of poor robustness of the algorithm, achieve the effect of improving segmentation results and reducing time-consuming
- Summary
- Abstract
- Description
- Claims
- Application Information
AI Technical Summary
Problems solved by technology
Method used
Image
Examples
Embodiment 2
[0073] According to an aspect of one or more embodiments of the present disclosure, there is provided a computer-readable storage medium.
[0074] A computer-readable storage medium, in which a plurality of instructions are stored, and the instructions are suitable for being loaded by a processor of a terminal device and executing the method for automatically segmenting multi-target organs at risk based on deep learning.
Embodiment 3
[0076] According to an aspect of one or more embodiments of the present disclosure, a terminal device is provided.
[0077] A terminal device, which includes a processor and a computer-readable storage medium, the processor is used to implement instructions; the computer-readable storage medium is used to store multiple instructions, and the instructions are suitable for being loaded by the processor and executing the described one A method for automatic segmentation of multi-target organs at risk based on deep learning.
[0078] These computer-executable instructions, when executed in a device, cause the device to perform the methods or processes described in accordance with various embodiments in the present disclosure.
[0079] In this embodiment, a computer program product may include a computer-readable storage medium carrying computer-readable program instructions for performing various aspects of the present disclosure. A computer readable storage medium may be a tangi...
Embodiment 4
[0083] According to an aspect of one or more embodiments of the present disclosure, a device for automatically segmenting multi-target organs at risk based on deep learning is provided.
[0084]A device for automatic segmentation of multi-target organs at risk based on deep learning, based on the described method for automatic segmentation of multi-target organs at risk based on deep learning, including:
[0085] a data acquisition module configured to receive patient input images;
[0086] The data conversion module is configured to convert the patient input image into JPEG format data;
[0087] The region of interest selection module is configured to input JPEG format data into the Overfeat location detection network trained according to the manual segmentation results of physicists, and automatically select regions of interest including multi-target organs at risk;
[0088] The contour inference module is configured to input the automatically selected region of interest in...
PUM
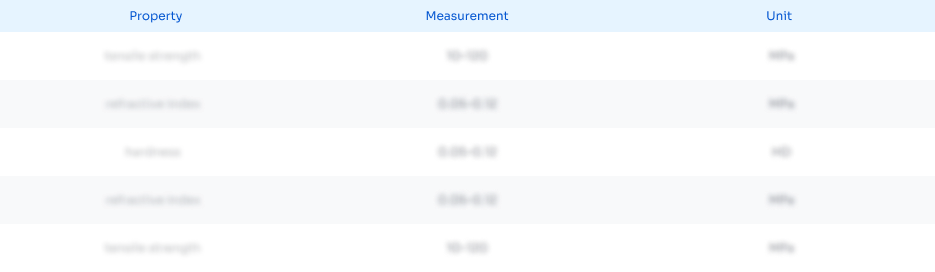
Abstract
Description
Claims
Application Information
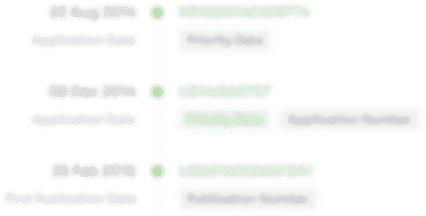
- R&D Engineer
- R&D Manager
- IP Professional
- Industry Leading Data Capabilities
- Powerful AI technology
- Patent DNA Extraction
Browse by: Latest US Patents, China's latest patents, Technical Efficacy Thesaurus, Application Domain, Technology Topic, Popular Technical Reports.
© 2024 PatSnap. All rights reserved.Legal|Privacy policy|Modern Slavery Act Transparency Statement|Sitemap|About US| Contact US: help@patsnap.com