Multi-domain image conversion method and system based on generative adversarial network
An image conversion and network technology, which is applied in the field of deep learning and can solve the problems of scarcity of multi-modal image data.
- Summary
- Abstract
- Description
- Claims
- Application Information
AI Technical Summary
Problems solved by technology
Method used
Image
Examples
Embodiment Construction
[0040] Such as figure 1 As shown, the implementation steps of the multi-domain image conversion method based on the generative confrontation network in this embodiment include:
[0041] 1) Input the original image x and original image y of the specified X and Y modes;
[0042] 2) Perform X-modal encoding on the original image x to obtain the first original image feature code_x, perform X-modal decoding on the first original image feature code_x to obtain the first reconstructed image x', and perform X-modal encoding on the first reconstructed image x' Encode to obtain the first reconstruction feature code_x'; perform Y-mode encoding on the original image y to obtain the second original image feature code_y, perform Y-mode decoding on the second original image feature code_y to obtain the second reconstruction image y', and convert the second reconstruction Figure y' is coded in Y mode to obtain the second reconstruction feature code_y';
[0043] 3) Perform feature identifica...
PUM
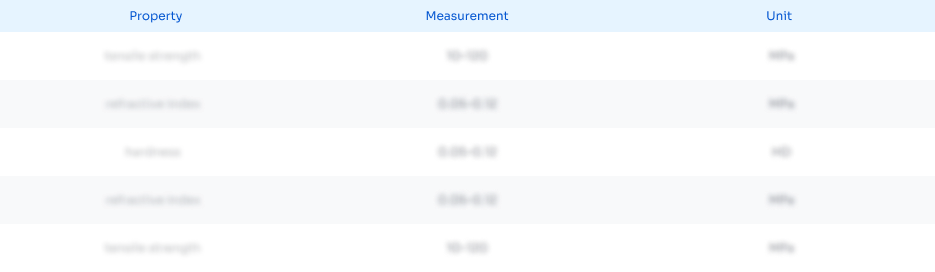
Abstract
Description
Claims
Application Information
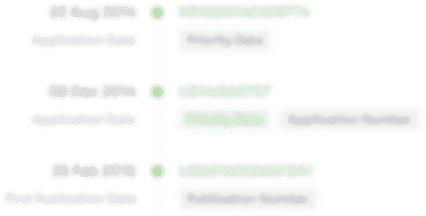
- R&D
- Intellectual Property
- Life Sciences
- Materials
- Tech Scout
- Unparalleled Data Quality
- Higher Quality Content
- 60% Fewer Hallucinations
Browse by: Latest US Patents, China's latest patents, Technical Efficacy Thesaurus, Application Domain, Technology Topic, Popular Technical Reports.
© 2025 PatSnap. All rights reserved.Legal|Privacy policy|Modern Slavery Act Transparency Statement|Sitemap|About US| Contact US: help@patsnap.com