Chopstick image classification method based on adaptive convolutional neural network
A convolutional neural network and classification method technology, applied in chopsticks image classification, chopsticks image classification based on adaptive convolutional neural network, can solve problems such as unqualified chopsticks that cannot be detected and smaller than set parameters, and improve quality , the effect of improving core competitiveness and high classification accuracy
- Summary
- Abstract
- Description
- Claims
- Application Information
AI Technical Summary
Problems solved by technology
Method used
Image
Examples
Embodiment 1
[0032]Chopsticks are not only China, but also the most important tableware in Asia. With the continuous development of the economy, people's requirements for diet and hygiene are constantly improving, so the requirements for tableware, especially chopsticks, are also gradually increasing. People not only require chopsticks to be durable and free from defects, but even engrave images on chopsticks, so the quality requirements for chopsticks are gradually increasing. In order to ensure food hygiene, the replacement frequency of chopsticks in normal families, restaurants and canteens is constantly increasing; with the vigorous development of the takeaway industry, the demand for disposable chopsticks has also grown exponentially; most of the traditional chopsticks are made of wood, bamboo, stainless steel and other materials, and with the continuous development of the manufacturing industry and the demand for environmental protection, many new materials are also used to make chop...
Embodiment 2
[0046] The chopsticks image classification method based on adaptive convolutional neural network is the same as embodiment 1, the construction initial adaptive convolutional neural network described in step (2), its structure is successively: the first convolutional layer→the first descending Sampling layer→second convolutional layer→second downsampling layer→third convolutional layer→third downsampling layer→fourth convolutional layer→fourth downsampling layer→fifth convolution Layer → fifth downsampling layer → first dropout layer → first fully connected layer.
[0047] The first ten layers of the twelve-layer initial adaptive convolutional neural network are composed of five interleaved convolutional layers and five downsampling layers. The five convolutional layers can fully guarantee the feature extraction capability of the network. Improve classification accuracy; five downsampling layers can fully compress the size of data, reduce the amount of calculation of the networ...
Embodiment 3
[0049] The chopstick image classification method based on the adaptive convolutional neural network is the same as that in Embodiment 1-2, and the parameters of each layer in the initial adaptive convolutional neural network constructed by the present invention can be changed according to the characteristics of the current chopsticks data set to include five kinds of defects Taking the bamboo chopsticks data set as an example, the parameters are set as follows:
[0050] The total number of input layer feature maps of the adaptive convolutional neural network is set to 3, and the feature map size is set to 80×500.
[0051] Set the total number of convolution filters in the first convolution layer to 32, the pixel size of the convolution filter to 3×3, the feature map size to 80×500, and the convolution step to 1 pixel.
[0052] The size of the pooling area in the first downsampling layer is set to 3×3, the pooling step is set to 3 pixels, and the feature map size is set to 27×1...
PUM
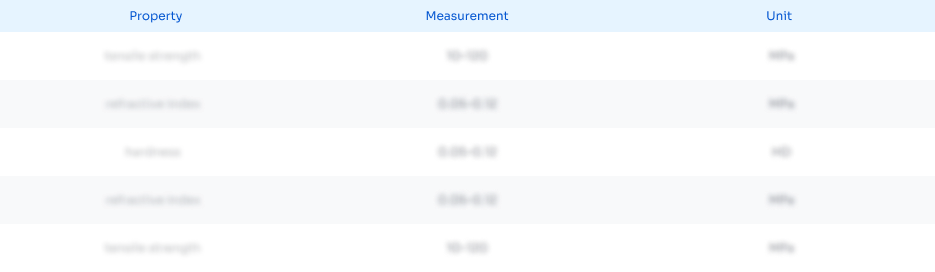
Abstract
Description
Claims
Application Information
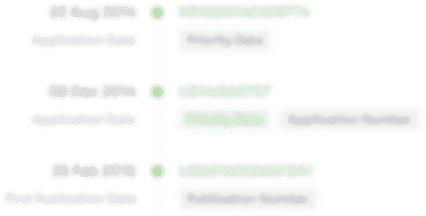
- R&D Engineer
- R&D Manager
- IP Professional
- Industry Leading Data Capabilities
- Powerful AI technology
- Patent DNA Extraction
Browse by: Latest US Patents, China's latest patents, Technical Efficacy Thesaurus, Application Domain, Technology Topic, Popular Technical Reports.
© 2024 PatSnap. All rights reserved.Legal|Privacy policy|Modern Slavery Act Transparency Statement|Sitemap|About US| Contact US: help@patsnap.com