Lithium battery remaining life prediction method
A technology of life prediction and lithium battery, which is applied in prediction, neural learning methods, and electrical measurement, can solve the problems of consuming training resources, low prediction accuracy, and difficulty in meeting work requirements, so as to improve computing efficiency, improve prediction accuracy, and effectively The effect of extraction and prediction
- Summary
- Abstract
- Description
- Claims
- Application Information
AI Technical Summary
Problems solved by technology
Method used
Image
Examples
Embodiment Construction
[0043] In order to make the object, technical solution and advantages of the present invention clearer, the present invention will be further described in detail below in conjunction with the accompanying drawings and embodiments. It should be understood that the specific embodiments described here are only used to explain the present invention, not to limit the present invention.
[0044] refer to figure 1 , a lithium battery life prediction method provided in an embodiment of the present invention, comprising the following steps:
[0045] (1) Collect the capacitance of multiple charge and discharge cycles of the lithium battery, and normalize the collected multiple capacitances;
[0046] Specifically, all collected capacitances are divided by the capacitance of the first charge-discharge cycle.
[0047] (2) Carry out window division to a plurality of electric capacity after normalization, and the electric capacity in each window and the next electric capacity of each windo...
PUM
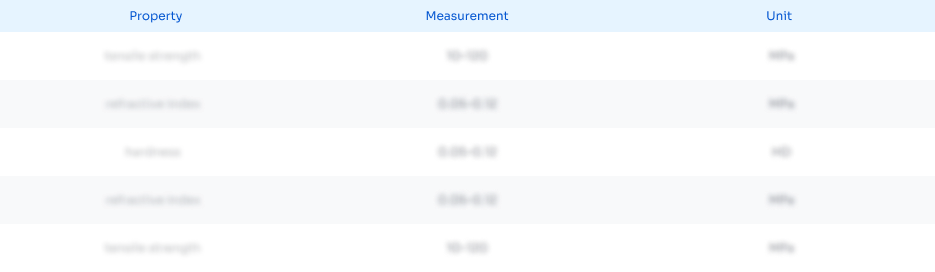
Abstract
Description
Claims
Application Information
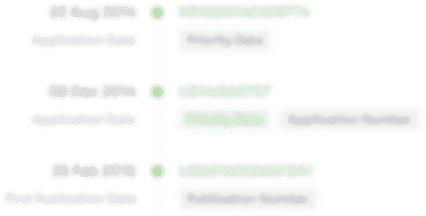
- R&D Engineer
- R&D Manager
- IP Professional
- Industry Leading Data Capabilities
- Powerful AI technology
- Patent DNA Extraction
Browse by: Latest US Patents, China's latest patents, Technical Efficacy Thesaurus, Application Domain, Technology Topic, Popular Technical Reports.
© 2024 PatSnap. All rights reserved.Legal|Privacy policy|Modern Slavery Act Transparency Statement|Sitemap|About US| Contact US: help@patsnap.com