Casting defect identification method based on convolutional neural network
A convolutional neural network and casting defect technology, which is applied in the field of casting defect recognition based on convolutional neural network, can solve the problems of low efficiency of manual detection, slow recognition speed, complicated storage and query process, etc., to improve efficiency and recognition. Accuracy, faster recognition, avoiding the effects of separate detection and management
- Summary
- Abstract
- Description
- Claims
- Application Information
AI Technical Summary
Problems solved by technology
Method used
Image
Examples
Embodiment Construction
[0025] In order to make the object, technical solution and advantages of the present invention clearer, the present invention will be further described in detail below in conjunction with the accompanying drawings and embodiments. It should be understood that the specific embodiments described here are only used to explain the present invention, not to limit the present invention. In addition, the technical features involved in the various embodiments of the present invention described below can be combined with each other as long as they do not constitute a conflict with each other.
[0026] figure 1 It is a flow chart of the casting defect recognition method based on the convolutional neural network constructed according to the preferred embodiment of the present invention, such as figure 1 As shown, a casting defect recognition method based on convolutional neural network, the method includes the following steps:
[0027] S1: Get the image dataset
[0028] Artificially s...
PUM
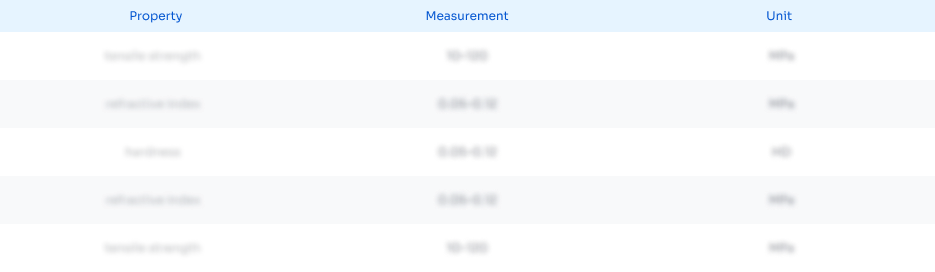
Abstract
Description
Claims
Application Information
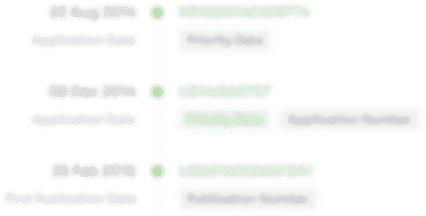
- R&D Engineer
- R&D Manager
- IP Professional
- Industry Leading Data Capabilities
- Powerful AI technology
- Patent DNA Extraction
Browse by: Latest US Patents, China's latest patents, Technical Efficacy Thesaurus, Application Domain, Technology Topic, Popular Technical Reports.
© 2024 PatSnap. All rights reserved.Legal|Privacy policy|Modern Slavery Act Transparency Statement|Sitemap|About US| Contact US: help@patsnap.com