Cigarette loose end rate prediction method and system based on improved gradient improvement decision tree
A forecasting method and decision tree technology, applied in forecasting, manufacturing computing systems, instruments, etc., can solve problems such as poor forecasting accuracy, omission, and slow convergence speed
- Summary
- Abstract
- Description
- Claims
- Application Information
AI Technical Summary
Problems solved by technology
Method used
Image
Examples
Embodiment 1
[0077] A method for predicting the short rate of cigarettes based on an improved gradient boosting decision tree, such as figure 1 shown, including the following steps:
[0078] S100. Obtain the process parameters of the same batch of shredded tobacco in each process of shredded tobacco and the short-short rate data in the final wrapping process, and form the original data set with the process parameters and short-short rate data;
[0079] S200. Divide the original data set based on the original data set combined with a correlation coefficient analysis method, determine key process parameters, and obtain key process parameter sets;
[0080] S300. Perform normalization processing on the data in the key process parameter set, and obtain a training data set and a testing data set by randomly dividing the normalized data;
[0081] S400. Based on the training samples in the training data set, establish a corresponding regression decision tree model through an iterative algorithm, ...
specific Embodiment
[0113] Based on the above steps, take the actual production data of a cigarette factory’s cigarette production line in 2018 as an example to illustrate the differences between this application and the prior art. First of all, communicate with the technical experts of the tobacco factory, and conduct correlation analysis on the accumulated massive production data, and obtain 10 key process parameters in the silk production process. According to the correlation analysis results, the values of these 10 process parameters are respectively As the input quantity of the model, the cigarette short rate under the final rolling process is used as the predictive variable of the model.
[0114] Tobacco cut production data is collected for a period of time, and the data set is preprocessed and analyzed to eliminate redundant process parameters and missing abnormal data of short rate. At the same time, the time information is used to realize the correspondence between the process paramete...
Embodiment 2
[0120] A cigarette short rate prediction system based on an improved gradient boosting decision tree, such as figure 2 As shown, it includes a data acquisition module 100, a preprocessing module 200, a reprocessing module 300, a model training module 400 and a prediction module 500;
[0121] The data acquisition module 100 is used to acquire the process parameters of the same batch of shredded tobacco in each process of shredded tobacco and the short-short rate data under the final wrapping process, and form the original data set with the process parameters and short-short rate data;
[0122] The preprocessing module 200 is used to divide the original data set based on the original data set combined with a correlation coefficient analysis method, determine key process parameters, and obtain key process parameter sets;
[0123] The reprocessing module 300 is configured to perform normalization processing on the data in the key process parameter set, and obtain a training data ...
PUM
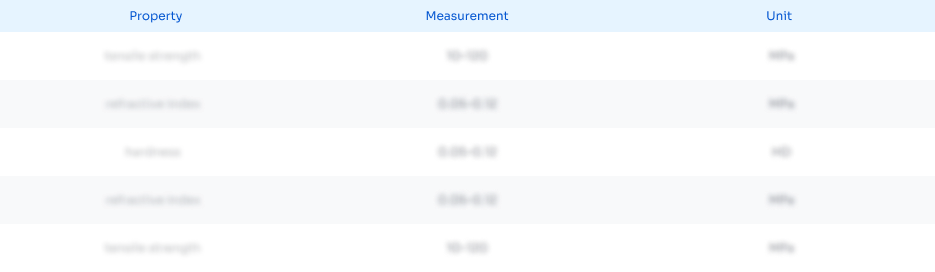
Abstract
Description
Claims
Application Information
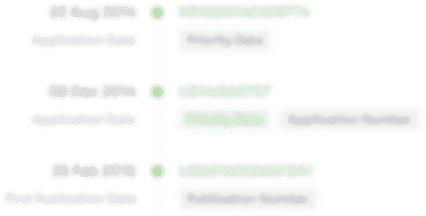
- R&D
- Intellectual Property
- Life Sciences
- Materials
- Tech Scout
- Unparalleled Data Quality
- Higher Quality Content
- 60% Fewer Hallucinations
Browse by: Latest US Patents, China's latest patents, Technical Efficacy Thesaurus, Application Domain, Technology Topic, Popular Technical Reports.
© 2025 PatSnap. All rights reserved.Legal|Privacy policy|Modern Slavery Act Transparency Statement|Sitemap|About US| Contact US: help@patsnap.com