Structure local defect detection method based on vector autoregression model
An autoregressive model and local defect technology, applied in measuring devices, measuring acceleration, speed/acceleration/impact measurement, etc., can solve the problems of small flaw detection area, difficult promotion, heavy workload, etc., and achieve simple calculation method and improved calculation Efficiency and applicability improvement effect
- Summary
- Abstract
- Description
- Claims
- Application Information
AI Technical Summary
Problems solved by technology
Method used
Image
Examples
Embodiment 1
[0058] Such as figure 1 As shown, the present invention relates to a method for identifying structural local defects based on a vector autoregressive model, which is realized by the following steps, including
[0059] Step 1: collecting the acceleration α response of the defective structure and the non-defective structure, and decomposing the collected acceleration α response into multiple samples with the same data length for the establishment of the subsequent vector autoregressive model (VAR);
[0060] Step 2: Use the AIC criterion to determine the order of the VAR model of each sample obtained in Step 1,
[0061] Step 3: According to the order of the VAR model determined in Step 2, establish a VAR model for each sample,
[0062] Step 4: Extract the diagonal elements of the coefficient matrix in each of the three VAR models in step 3, and define a new eigenvector f,
[0063] Step 5: According to the vector f in step 4, respectively calculate the Mahalanobis distance of th...
Embodiment 2
[0089] In step 2 of Embodiment 1, the order of the VAR model can be determined according to the AIC criterion can be replaced by the BIC criterion. BIC (Bayesian Information Criterion) Bayesian Information Criterion is similar to AIC and is used for model selection. It was proposed by Schwarz in 1978. When training the model, increasing the number of parameters, that is, increasing the complexity of the model, will increase the likelihood function, but it will also lead to overfitting. To solve this problem, both AIC and BIC introduce a penalty item related to the number of model parameters. , the penalty term of BIC is larger than that of AIC, considering the number of samples. When the number of samples is too large, it can effectively prevent the model complexity from being too high due to high model accuracy.
[0090] BIC=kln(n)-2ln(L)
[0091] Among them, k is the number of model parameters, n is the number of samples, and L is the likelihood function. The kln(n) penalt...
Embodiment 3
[0093] The CUSUM control chart method in Step 6 of Embodiment 1 can be replaced by EWMA.
[0094] EWMA (Weighted Moving Average) is a type of time-weighted control chart in which an exponentially weighted moving average is plotted. Each EWMA point combines information from all previous subgroups or observations according to a user-defined weighting factor. The advantage of EWMA charts is that they are not heavily affected when small or large values enter the calculation. By varying the weights used and the number of s to control the limit, a control chart can be constructed that can detect shifts in the process of almost any size. For this reason, EWMA control charts are often used to monitor controlled processes to detect small excursions from the target. Its EWMA statistic Z is calculated as follows:
[0095] Z 0 =λMD 1
[0096] Z j =λMD j+1 +(1-λ)MD j-1 , 0.05≤λ≤0.25
PUM
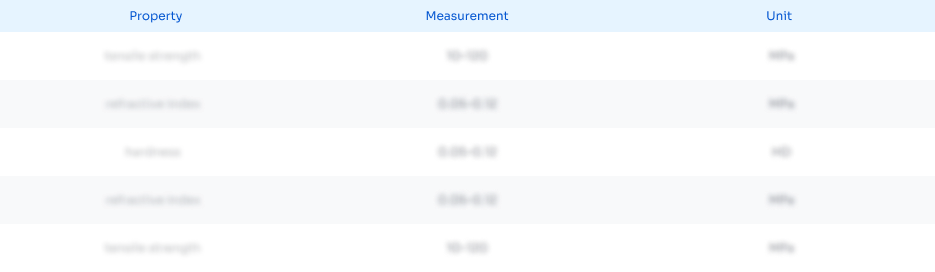
Abstract
Description
Claims
Application Information
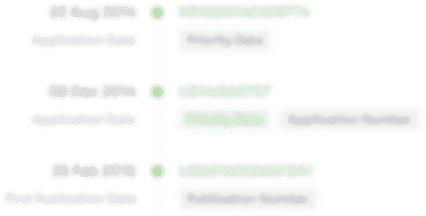
- R&D Engineer
- R&D Manager
- IP Professional
- Industry Leading Data Capabilities
- Powerful AI technology
- Patent DNA Extraction
Browse by: Latest US Patents, China's latest patents, Technical Efficacy Thesaurus, Application Domain, Technology Topic, Popular Technical Reports.
© 2024 PatSnap. All rights reserved.Legal|Privacy policy|Modern Slavery Act Transparency Statement|Sitemap|About US| Contact US: help@patsnap.com