Vehicle model identification method based on convolutional neural network
A convolutional neural network and vehicle recognition technology, applied in biological neural network models, neural learning methods, neural architectures, etc., can solve the problems of blurred vehicle recognition lighting images, low vehicle recognition accuracy, and impact on vehicle recognition accuracy. Improve vehicle recognition accuracy, improve efficiency, and reduce the effect of overfitting
- Summary
- Abstract
- Description
- Claims
- Application Information
AI Technical Summary
Problems solved by technology
Method used
Image
Examples
Embodiment Construction
[0053] The specific implementation manners of the present invention will be further described in detail below in conjunction with the accompanying drawings and embodiments. The following examples are used to illustrate the present invention, but are not intended to limit the scope of the present invention.
[0054] Such as figure 1 As shown, the method of this embodiment is as follows.
[0055] Step 1: Collect the car model image data set, use data enhancement to amplify the data set to obtain a picture that is closer to the real scene, and divide the amplified data set into a training set and a test set according to a certain proportion;
[0056] The car model picture data set used in this embodiment is a BIT-vehicle data set, with a total of 9850 pictures, including 6 types of passenger cars, vans, micro cars, cars, SUVs and trucks;
[0057] Among them, two strategies of rotation and Gaussian blur are used to process each image in the BIT-vehicle model image data set, and th...
PUM
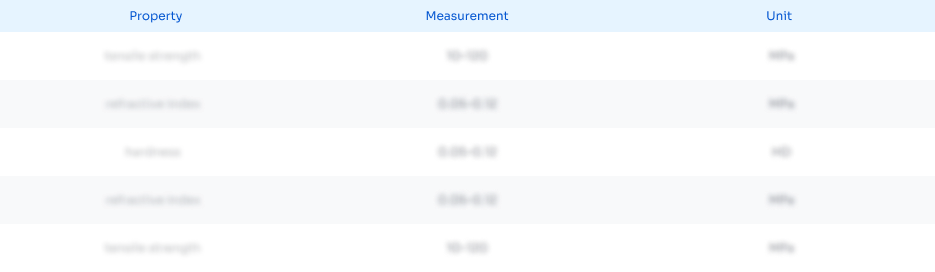
Abstract
Description
Claims
Application Information
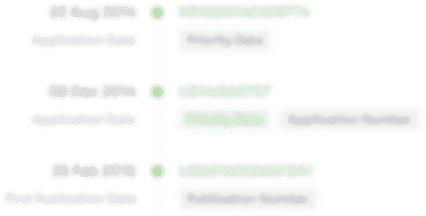
- R&D
- Intellectual Property
- Life Sciences
- Materials
- Tech Scout
- Unparalleled Data Quality
- Higher Quality Content
- 60% Fewer Hallucinations
Browse by: Latest US Patents, China's latest patents, Technical Efficacy Thesaurus, Application Domain, Technology Topic, Popular Technical Reports.
© 2025 PatSnap. All rights reserved.Legal|Privacy policy|Modern Slavery Act Transparency Statement|Sitemap|About US| Contact US: help@patsnap.com