Collision avoidance planning method for mobile robot based on deep reinforcement learning in dynamic environment
A mobile robot, dynamic environment technology, applied in neural learning methods, instruments, electromagnetic wave re-radiation and other directions, can solve the problems of unsuitable dynamic unknown environment, large limitations, etc., to achieve adaptable high efficiency, reduce difficulty, The effect of improving the success rate of obstacle avoidance
- Summary
- Abstract
- Description
- Claims
- Application Information
AI Technical Summary
Problems solved by technology
Method used
Image
Examples
Embodiment Construction
[0041] The present invention will be further described below with reference to the accompanying drawings and cases.
[0042] The invention discloses a collision avoidance planning method for a mobile robot based on deep reinforcement learning in a dynamic environment, belongs to the technical field of mobile robot navigation, and can be used for effective obstacle avoidance when a mobile robot works in a multi-type dynamic obstacle environment. The invention collects the original data through a laser range finder, uses the original data as the input of the neural network after corresponding processing, establishes the LSTM neural network, outputs the corresponding parameters through the A3C algorithm, and obtains the action of each step of the robot through processing. The flow chart of the overall obstacle avoidance algorithm is as follows figure 1 shown. The present invention does not need to model the environment, is more suitable for unknown obstacle environment, adopts a...
PUM
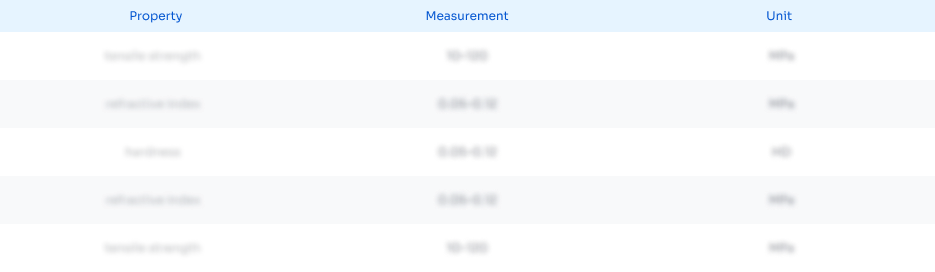
Abstract
Description
Claims
Application Information
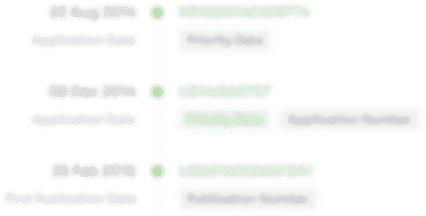
- R&D
- Intellectual Property
- Life Sciences
- Materials
- Tech Scout
- Unparalleled Data Quality
- Higher Quality Content
- 60% Fewer Hallucinations
Browse by: Latest US Patents, China's latest patents, Technical Efficacy Thesaurus, Application Domain, Technology Topic, Popular Technical Reports.
© 2025 PatSnap. All rights reserved.Legal|Privacy policy|Modern Slavery Act Transparency Statement|Sitemap|About US| Contact US: help@patsnap.com