Cascade target recognition method and system based on deep learning
A deep learning and target recognition technology, applied in neural learning methods, character and pattern recognition, instruments, etc., can solve problems such as poor handling and poor detection of small targets, and achieve the effect of reducing interference and improving detection accuracy.
- Summary
- Abstract
- Description
- Claims
- Application Information
AI Technical Summary
Problems solved by technology
Method used
Image
Examples
Embodiment 1
[0041] In the first embodiment, an image of power transmission and transformation is used as an input, and the target is a defect of power transmission and transformation equipment, which is described as an example.
[0042] In the cascade target recognition method based on deep learning figure 1 Showcasing the cascaded detection structure of the predictive architecture, figure 2 Shows the structure of the parallel target detection logic reasoning mechanism for image preprocessing, image 3 It shows the logic reasoning mechanism structure of nth level model parallel object detection.
[0043] The specific method includes the following steps:
[0044] In the example of the present disclosure, the power transmission line image of the UAV is used as the sample source. UAV is used to collect images of typical transmission line defects.
[0045] The disclosed example only takes the component defect at the bolt and pin of the sample defect category as an example. Manual select...
Embodiment 2
[0064] The difference from the above-mentioned embodiment is that the input data of this embodiment is the pre-determined regional inspection image collected by the drone, and the recognition target to be recognized is a regional disaster (such as debris flow, fire, etc.).
[0065] Obtain the inspection image of the area to be observed, and perform image preprocessing. The preprocessing methods include image rotation, image mirroring, image defogging, image enhancement, image deblurring and other methods.
[0066] Perform image augmentation.
[0067] Image expansion can choose to traverse and use all image processing methods for sample expansion, or use random probability to select image processing methods for sample expansion.
PUM
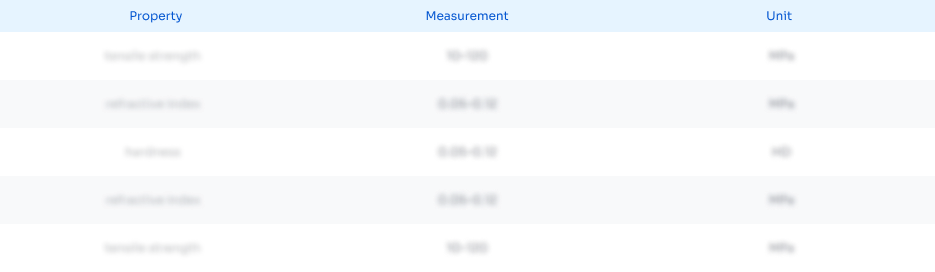
Abstract
Description
Claims
Application Information
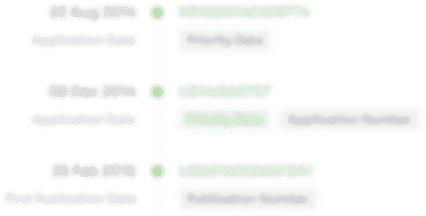
- R&D Engineer
- R&D Manager
- IP Professional
- Industry Leading Data Capabilities
- Powerful AI technology
- Patent DNA Extraction
Browse by: Latest US Patents, China's latest patents, Technical Efficacy Thesaurus, Application Domain, Technology Topic.
© 2024 PatSnap. All rights reserved.Legal|Privacy policy|Modern Slavery Act Transparency Statement|Sitemap