Video human body behavior recognition method and system based on multi-mode double-flow 3D network
A recognition method and multi-modal technology, applied in character and pattern recognition, biological neural network models, instruments, etc., can solve problems such as difficult to obtain high-level clues, and achieve the effect of eliminating interference
- Summary
- Abstract
- Description
- Claims
- Application Information
AI Technical Summary
Problems solved by technology
Method used
Image
Examples
Embodiment 1
[0029] In one or more embodiments, a video human behavior recognition method based on a multimodal dual-stream 3D network is disclosed, such as figure 1 shown, including the following steps:
[0030] (1) For an action sample, synchronized RGB and depth videos are collected separately.
[0031] (2) Depth dynamic image sequence generated based on depth video;
[0032] (3) Posture evaluation map sequence generated based on RGB video;
[0033] (4) Input the depth dynamic map sequence and the pose evaluation map sequence into the 3D convolutional neural network respectively, and obtain the respective classification results;
[0034] (5) The obtained classification results are fused to obtain the final behavior recognition result.
[0035] In this implementation method, the depth dynamic image sequence DDIS and the pose evaluation image sequence PEMS are respectively used as the data input of two 3DCNNs in the framework, and the DDIS stream and the PEMS stream are constructed. Th...
PUM
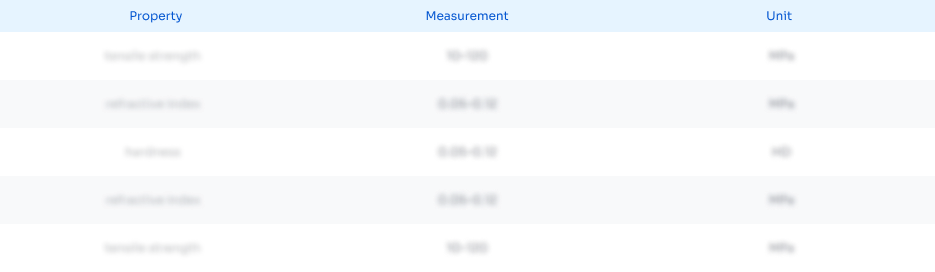
Abstract
Description
Claims
Application Information
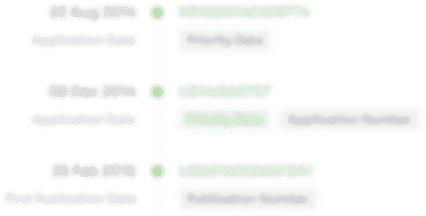
- R&D Engineer
- R&D Manager
- IP Professional
- Industry Leading Data Capabilities
- Powerful AI technology
- Patent DNA Extraction
Browse by: Latest US Patents, China's latest patents, Technical Efficacy Thesaurus, Application Domain, Technology Topic, Popular Technical Reports.
© 2024 PatSnap. All rights reserved.Legal|Privacy policy|Modern Slavery Act Transparency Statement|Sitemap|About US| Contact US: help@patsnap.com