A deep learning-based image recognition method for leaf diseases of medicinal plants
A technology of medicinal plants and deep learning, applied in neural learning methods, image enhancement, image data processing, etc., can solve the problems of small amount of sample data, low recognition accuracy, and large number of convolution kernel parameters
- Summary
- Abstract
- Description
- Claims
- Application Information
AI Technical Summary
Problems solved by technology
Method used
Image
Examples
Embodiment 1
[0044] Please refer to figure 1 , figure 2 , image 3 and Figure 4 , an embodiment of the present invention provides a deep learning-based image recognition method for leaf diseases of medicinal plants, comprising the following steps:
[0045] S1. Collect several images of leaf diseases of medicinal plants, and rename each image in the form of plant name + disease name;
[0046] S2, performing enhancement processing on the renamed image of leaf diseases of medicinal plants;
[0047] S3, image data preprocessing, and uniformly adjust the size of the images of leaf diseases of medicinal plants after each enhancement processing to 299x299;
[0048] S4. Train the deep CNN model. The deep CNN model includes the convolution pooling network in series, the Inception-I network, the average pooling network, the Dropout layer and the Softmax layer. The last two convolution layers of the convolution pooling network in series are Depthwise separable convolutional layers, including ran...
Embodiment 2
[0064] For step S1 in Example 1, images of leaf diseases of medicinal plants are collected by a digital camera, and there are 500 images of leaf diseases of medicinal plants in total.
Embodiment 3
[0066] For step S2 in Embodiment 1, the enhancement processing on the leaf disease image includes image rotation, mirror symmetry, brightness adjustment and PCA jitter.
[0067] In this embodiment, image rotation refers to rotating all pixels of the image by an angle of 0-360 degrees around the center of the image; mirror symmetry refers to using the mid-perpendicular line in the image as the axis, and exchanging all the pixels in the image is horizontal symmetry . Set the coordinates of any point P in the image to be (x0, y0), and the coordinates after rotating θ degrees counterclockwise are (x, y), then the calculation formula of the polar coordinates before and after the rotation is as follows:
[0068] x0=γcosα, y0=γsinα
[0069] x=γcos(α+θ), y=γsin(α+θ)
[0070] Where γ represents the polar diameter of point P; α represents the polar angle of point P.
[0071] In this embodiment, the brightness adjustment refers to adjusting the sharpness value, brightness value and co...
PUM
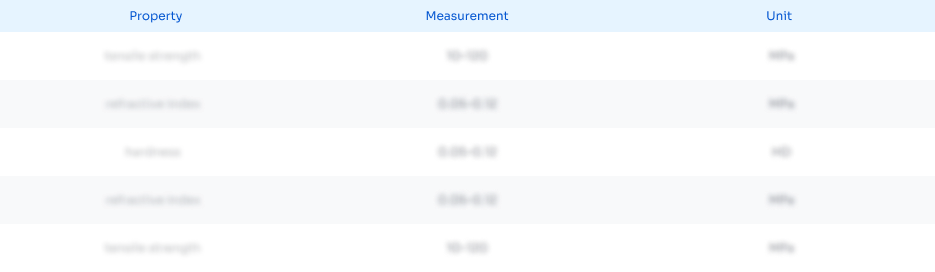
Abstract
Description
Claims
Application Information
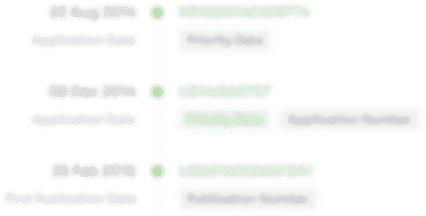
- R&D Engineer
- R&D Manager
- IP Professional
- Industry Leading Data Capabilities
- Powerful AI technology
- Patent DNA Extraction
Browse by: Latest US Patents, China's latest patents, Technical Efficacy Thesaurus, Application Domain, Technology Topic, Popular Technical Reports.
© 2024 PatSnap. All rights reserved.Legal|Privacy policy|Modern Slavery Act Transparency Statement|Sitemap|About US| Contact US: help@patsnap.com