Human body behavior recognition method of non-local double-flow convolutional neural network model
A convolutional neural network and recognition method technology, applied in the field of computer vision image and video processing, can solve problems such as low recognition accuracy, complex background environment, and diverse human behaviors, achieve good training results, and alleviate network overfitting , Good classification and recognition effect
- Summary
- Abstract
- Description
- Claims
- Application Information
AI Technical Summary
Problems solved by technology
Method used
Image
Examples
Embodiment Construction
[0018] The invention provides a human body behavior recognition method of a non-local dual-stream convolutional neural network model, the overall network structure adopts a dual-stream convolutional neural network model, figure 1 It is briefly explained that the network framework mainly includes spatial stream and temporal stream CNN models, which are used to extract spatial appearance information and temporal motion information of video samples. The structure and settings of each convolutional layer and fully connected layer are the same and the weight parameters are shared.
[0019] figure 1 The input video sample set is preprocessed to obtain RGB frames and optical flow images (single RGB frame and continuous optical flow frame), which are divided into training set and test set, which are respectively sent to spatial stream CNN and temporal stream CNN for training and testing. Select an appropriate size input for the RGB frame and optical flow image, select an appropriate ...
PUM
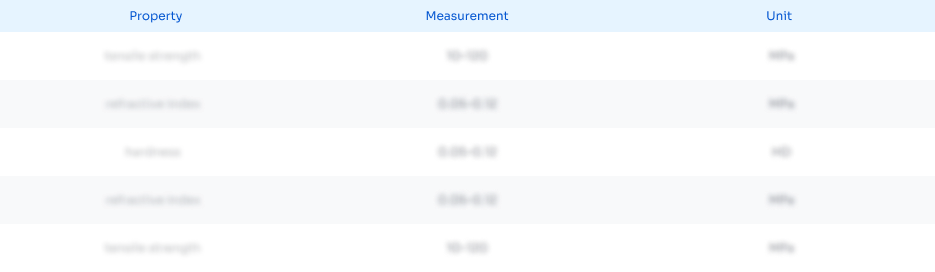
Abstract
Description
Claims
Application Information
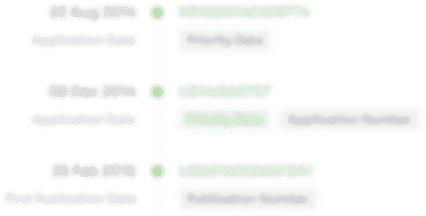
- R&D
- Intellectual Property
- Life Sciences
- Materials
- Tech Scout
- Unparalleled Data Quality
- Higher Quality Content
- 60% Fewer Hallucinations
Browse by: Latest US Patents, China's latest patents, Technical Efficacy Thesaurus, Application Domain, Technology Topic, Popular Technical Reports.
© 2025 PatSnap. All rights reserved.Legal|Privacy policy|Modern Slavery Act Transparency Statement|Sitemap|About US| Contact US: help@patsnap.com