Classifier training method and computer readable storage medium
A training method and classifier technology, applied in the field of classifier training, can solve the problems of low classification results, narrow adaptability, large detection accuracy error, etc., so as to reduce the time required for training, improve the classification effect, and improve the extraction time. Effect
- Summary
- Abstract
- Description
- Claims
- Application Information
AI Technical Summary
Problems solved by technology
Method used
Image
Examples
Embodiment 1
[0061] In the embodiment proposed by the present invention, such as figure 1 As shown, the training methods of the classifier include:
[0062] Step S102, decomposing the time series of EEG signals containing cold pain information to obtain decomposed subband components;
[0063] Step S104, determining the ratio of the energy value of any subband component to the sum of the energy values of all subband components, the fine compound multiscale dispersion entropy of any subband component, the fine compound multiscale fuzzy entropy and the autoregressive model coefficient;
[0064] Step S106, determining the feature set according to the ratio of the energy value of any subband component to the sum of the energy values of all subband components, fine composite multiscale dispersion entropy, fine composite multiscale fuzzy entropy, and autoregressive model coefficients;
[0065] Step S108, train the classifier according to the feature set to obtain the target classifier.
[0...
Embodiment 2
[0143] In the above embodiment, further comprising: performing dimensionality reduction on the feature set.
[0144] In this embodiment, by reducing the dimensionality of the feature set, the problem of large amount of calculation, calculation time, and excessive resource consumption caused by too many feature dimensions is eliminated, and the impact of too many feature dimensions on feature detection and feature detection is reduced. Classification effects.
[0145] Such as Figure 6 As shown, use a feature dimensionality reduction algorithm for dimensionality reduction, such as PCA (Principal components analysis, principal component analysis), to remove redundant features, and its specific steps include:
[0146] Step 1, get n-dimensional sample set D={x (1) , x (2) ,...x (m)};
[0147] Step 2, centralize all samples: Form a new data set X={s (1) ,s (2) ,...,s (m)};
[0148] Step 3, calculate the covariance matrix XX of the sample T ;
[0149] Step 4, for matrix...
Embodiment 3
[0154] In any of the above embodiments, it also includes: determining at least one of the sensitivity, specificity, positive predictive value and accuracy of the classifier; and according to at least one of the sensitivity, specificity, positive predictive value and accuracy A selection target classifier.
[0155] In this embodiment, the classifier is evaluated by introducing one or more evaluation indicators in sensitivity, specificity, positive predictive value and accuracy, and the target classifier is selected according to the evaluation results, so as to obtain the optimal classifier , so as to ensure the classification accuracy of the classifier.
[0156] Among them, the calculation formulas of sensitivity, specificity, positive predictive value and accuracy are as follows:
[0157]
[0158]
[0159]
[0160]
[0161] Among them, TP means true positive; TN means true negative; FP means false positive; FN means false negative.
PUM
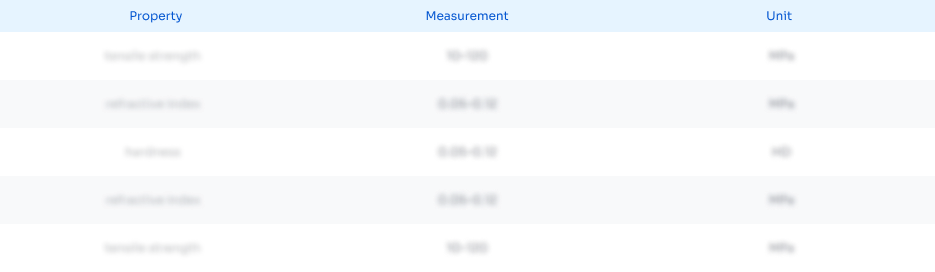
Abstract
Description
Claims
Application Information
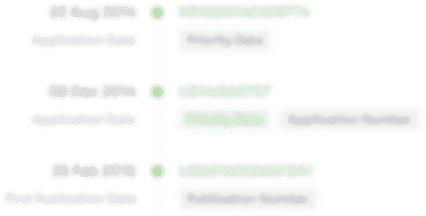
- Generate Ideas
- Intellectual Property
- Life Sciences
- Materials
- Tech Scout
- Unparalleled Data Quality
- Higher Quality Content
- 60% Fewer Hallucinations
Browse by: Latest US Patents, China's latest patents, Technical Efficacy Thesaurus, Application Domain, Technology Topic, Popular Technical Reports.
© 2025 PatSnap. All rights reserved.Legal|Privacy policy|Modern Slavery Act Transparency Statement|Sitemap|About US| Contact US: help@patsnap.com