Behavior recognition system and method based on multi-convolution kernel residual network, medium and equipment
A recognition system and multi-convolution technology, applied in the field of artificial intelligence and pattern recognition, can solve the problems of difficult to extract data related features and large correlation, and achieve the effect of enhancing feature extraction ability, comprehensiveness and feature extraction ability.
- Summary
- Abstract
- Description
- Claims
- Application Information
AI Technical Summary
Problems solved by technology
Method used
Image
Examples
Embodiment 1
[0063] An action recognition system based on multi-convolution kernel residual network and adaptive weight cross-entropy loss function, such as figure 1 As shown, it includes data preparation layer, deep learning layer and recognition application layer connected in sequence.
[0064] The data preparation layer collects relevant human motion data, human physiological data, environmental state data, etc. through sensors deployed on the human body or in the environment, and uploads the data to the cloud server through wireless transmission technology, and performs data processing on the data on the server. Cleaning, data alignment, missing value processing, standardization, normalization and other preprocessing operations and data labeling, and finally transfer the data to the deep learning layer.
[0065] The deep learning layer transmits the data input by the above-mentioned data preparation layer to the deep learning model based on the multi-convolution kernel residual network...
Embodiment 2
[0082] An action recognition method based on multi-convolution kernel residual network and adaptive weight cross-entropy loss function, such as figure 2 As shown, taking the identification of 17 behaviors in the OPPTUNITY dataset as an example, the sample data is learned through the multi-convolution kernel residual network, and a deep learning model is generated to identify the 17 behaviors. The specific steps include:
[0083] Step S01: Data preprocessing:
[0084]Perform data cleaning, data alignment, missing value processing, data up / down sampling, standardization / normalization and other preprocessing operations on the data in the OPPTUNITY dataset. Data cleaning is to clean and delete data that cannot be corrected due to transmission errors. Alignment is to eliminate the problem of inconsistent receiving time of some data due to wireless propagation delay. Missing value processing is to solve the problem of missing some data due to packet loss in data transmission. Data ...
Embodiment 3
[0132] A computer-readable storage medium, in which a plurality of instructions are stored, and the instructions are suitable for being loaded by a processor of a terminal device and executing the behavior recognition method based on a multi-convolution kernel residual network given in Embodiment 2. step.
PUM
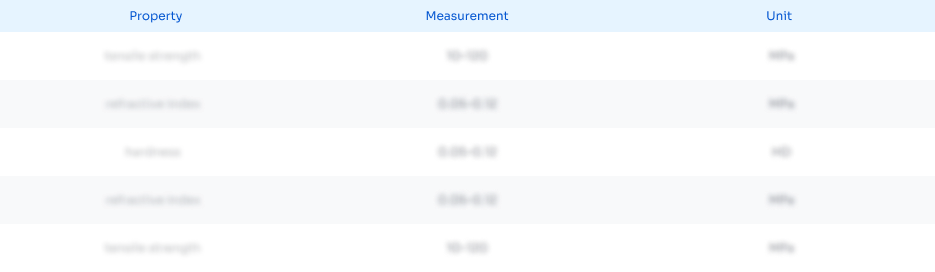
Abstract
Description
Claims
Application Information
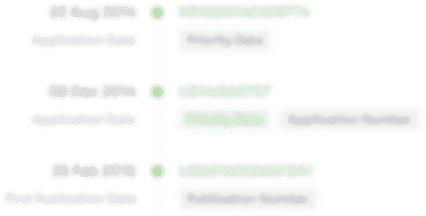
- R&D Engineer
- R&D Manager
- IP Professional
- Industry Leading Data Capabilities
- Powerful AI technology
- Patent DNA Extraction
Browse by: Latest US Patents, China's latest patents, Technical Efficacy Thesaurus, Application Domain, Technology Topic, Popular Technical Reports.
© 2024 PatSnap. All rights reserved.Legal|Privacy policy|Modern Slavery Act Transparency Statement|Sitemap|About US| Contact US: help@patsnap.com