Wind power plant short-term wind power prediction modeling method based on wavelet analysis and multi-model AdaBoost deep network
A wavelet analysis and deep network technology, applied in prediction, character and pattern recognition, instruments, etc., can solve problems such as inability to apply, insufficient generalization ability of feedforward neural network in wind power data, and inability to effectively obtain data with time series structure. , to achieve the effect of reducing dimension, improving convergence and enhancing uncertainty
- Summary
- Abstract
- Description
- Claims
- Application Information
AI Technical Summary
Problems solved by technology
Method used
Image
Examples
Embodiment Construction
[0043] Improving the prediction accuracy of wind power in wind farms can effectively reduce the huge impact on the stable operation of the grid when intermittent power sources with high permeability are connected to the grid, and at the same time can improve the system's ability to absorb new energy grid-connected. One of the difficulties in wind power forecasting modeling is to infer the hidden laws from limited samples and to identify unknown systems from experimental data. The inherent randomness, intermittentness and volatility of wind power increase the difficulty of short-term wind power forecasting.
[0044] The invention relates to a multi-scale resolution decomposition of wind speed using a two-dimensional wavelet to reduce the effects of instantaneity, randomness and uncertainty in wind speed, and then use a multi-model AdaBoost deep network to improve neural network integration and ease Falling into the defects of local minimums, enhancing the adaptability to different...
PUM
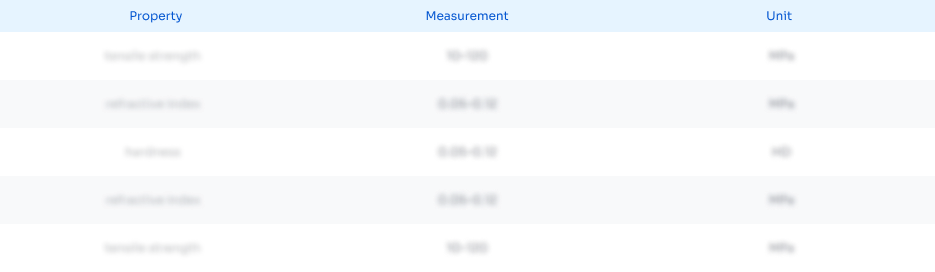
Abstract
Description
Claims
Application Information
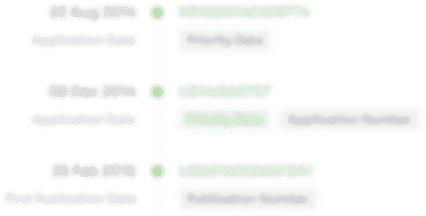
- R&D Engineer
- R&D Manager
- IP Professional
- Industry Leading Data Capabilities
- Powerful AI technology
- Patent DNA Extraction
Browse by: Latest US Patents, China's latest patents, Technical Efficacy Thesaurus, Application Domain, Technology Topic, Popular Technical Reports.
© 2024 PatSnap. All rights reserved.Legal|Privacy policy|Modern Slavery Act Transparency Statement|Sitemap|About US| Contact US: help@patsnap.com