Unbalanced hard disk data fault diagnosis method based on deep learning
A technology of deep learning and fault diagnosis, applied in faulty hardware testing methods, machine learning, electrical digital data processing, etc., can solve problems such as unbalanced data sets, achieve high robustness, low environmental requirements, and strong noise resistance Effect
- Summary
- Abstract
- Description
- Claims
- Application Information
AI Technical Summary
Problems solved by technology
Method used
Image
Examples
Embodiment
[0043] Since the hard disk model is specified by the manufacturer, the present invention trains different models according to different models. Capacity_bytes is the capacity of each hard disk, and S.M.A.R.T. numbers are some self-monitoring analysis and reporting techniques, which can represent the characteristics of the hard disk. The hard drive data is distributed in the form of dates, and more importantly, each hard drive has nearly 120 features, and in each feature, the missing values are half of the total. On this basis, the present invention adopts the three-dimensional reconstruction of the original data, and preprocesses the data features with the feature engineering Auto-encoder and the missing value processing method. The present invention adopts the sample division method for extremely unbalanced samples , the processed training set is processed by SMOTE to balance the number of unbalanced samples, and finally a model with higher accuracy is obtained by combining ...
PUM
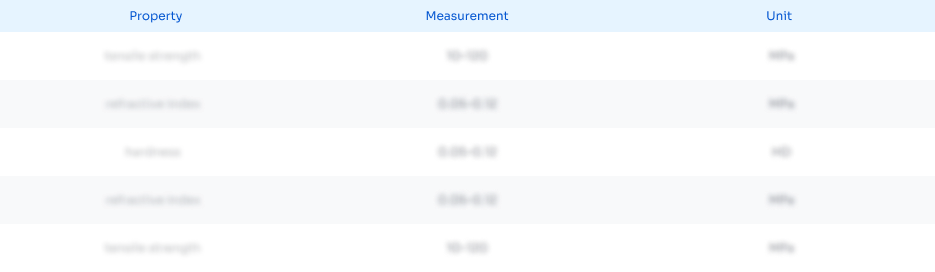
Abstract
Description
Claims
Application Information
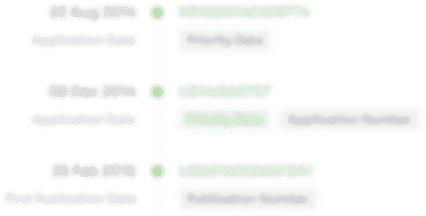
- R&D
- Intellectual Property
- Life Sciences
- Materials
- Tech Scout
- Unparalleled Data Quality
- Higher Quality Content
- 60% Fewer Hallucinations
Browse by: Latest US Patents, China's latest patents, Technical Efficacy Thesaurus, Application Domain, Technology Topic, Popular Technical Reports.
© 2025 PatSnap. All rights reserved.Legal|Privacy policy|Modern Slavery Act Transparency Statement|Sitemap|About US| Contact US: help@patsnap.com