The method is suitable for typical power utilization mode extraction method of massive types of unbalanced load data
A technology for balancing loads and power consumption patterns, applied in data processing applications, special data processing applications, neural learning methods, etc., can solve the imbalance of load data categories, excessive training samples, and the impact of models on the ability to distinguish minority samples, etc. problem, to achieve the effect of improving classification accuracy, high computing efficiency, and strong time series modeling ability
- Summary
- Abstract
- Description
- Claims
- Application Information
AI Technical Summary
Problems solved by technology
Method used
Image
Examples
Embodiment
[0039] Such as Figure 1 to Figure 4 As shown in , a typical power consumption pattern extraction method suitable for massive unbalanced load data includes the following steps:
[0040] (S1) Use the Borderline-SMOTE training sample category imbalance processing method to process the load data; the Borderline-SMOTE training sample category imbalance processing method first finds out the minority class training samples that are similar to the majority class according to the Euclidean distance between the load curves. For the adjacent boundary elements, the SMOTE algorithm is used to randomly synthesize new training samples for the boundary set, and the data synthesis ratio is adjusted to roughly balance the number of samples in the majority class and the minority class. The specific steps of the Borderline-SMOTE training sample category imbalance processing method are as follows:
[0041] (S11) Calculate each sample point p in the minority class P in the entire training set T ...
PUM
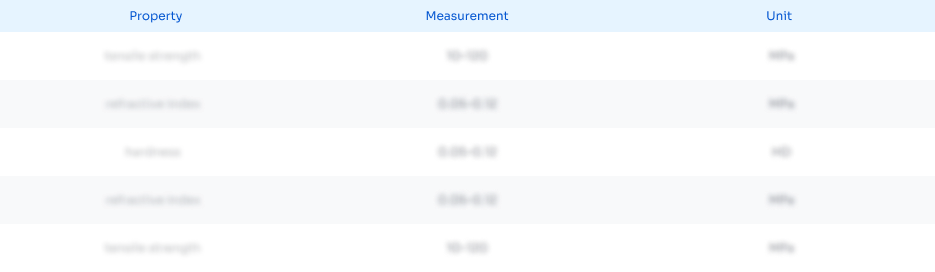
Abstract
Description
Claims
Application Information
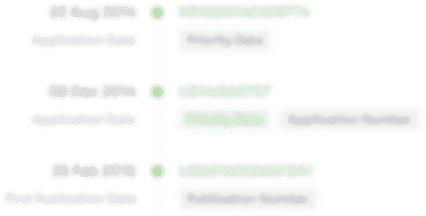
- R&D Engineer
- R&D Manager
- IP Professional
- Industry Leading Data Capabilities
- Powerful AI technology
- Patent DNA Extraction
Browse by: Latest US Patents, China's latest patents, Technical Efficacy Thesaurus, Application Domain, Technology Topic.
© 2024 PatSnap. All rights reserved.Legal|Privacy policy|Modern Slavery Act Transparency Statement|Sitemap