Semi-supervised text classification model training method, text classification method, system, device and medium
A text classification and model training technology, applied in the field of deep learning, can solve the problems of not being able to use text classification directly, affecting the accuracy of the training model, and not considering the confidence of the model, so as to alleviate the lack of problems, avoid the impact, and improve the performance.
- Summary
- Abstract
- Description
- Claims
- Application Information
AI Technical Summary
Problems solved by technology
Method used
Image
Examples
Embodiment 1
[0068] This embodiment provides a semi-supervised text classification model training method, such as figure 1 As shown, the method includes the following steps:
[0069] S101. Obtain an initial sample set, where the initial sample set includes a labeled sample set and the unlabeled sample set where x i Indicates the i-th labeled sample, u i Represents the i-th unlabeled sample, n represents the number of labeled samples, and m represents the number of unlabeled samples. In this embodiment, a labeled sample refers to a sample labeled with a classification label, and an unlabeled sample refers to a sample not labeled with a classification label.
[0070] S102, mark each sample x i and unlabeled sample u i Perform data cleaning. For example, suppose it is necessary to train a text classification model for a certain language (such as Chinese), then delete the words in the sample that are not in the language. In addition, cleaning processing such as stop word filtering ca...
Embodiment 2
[0110] This embodiment provides a text classification method, such as figure 2 shown, including the following steps:
[0111] S201, acquiring the target text to be classified;
[0112] S202, input the target text into the target text classification model trained according to the aforementioned text classification model training method for processing, obtain the predicted probability that the target text belongs to each classification label, and use the classification label corresponding to the maximum value of the prediction probability as the target text classification results.
[0113] Since the accuracy of the target text classification model trained according to the foregoing text classification model training method is high, the classification result obtained in this embodiment is more accurate.
Embodiment 3
[0115] This embodiment provides a semi-supervised text classification model training system, such as image 3 As shown, the system 10 includes: an initial sample set acquisition module 101, a cleaning module 102, an enhancement module 103, a model processing module 104, a new sample construction module 105, a label estimation module 106, a verification module 107, and a trusted sample acquisition module 108 , a confidence sample set construction module 109 , an expansion module 110 and a model training module 111 . Each module is described in detail below:
[0116] The initial sample set obtaining module 101 is used to obtain the initial sample set, and the initial sample set includes the labeled sample set and the unlabeled sample set where x i Indicates the i-th labeled sample, u i Represents the i-th unlabeled sample, n represents the number of labeled samples, and m represents the number of unlabeled samples. In this embodiment, a labeled sample refers to a sample l...
PUM
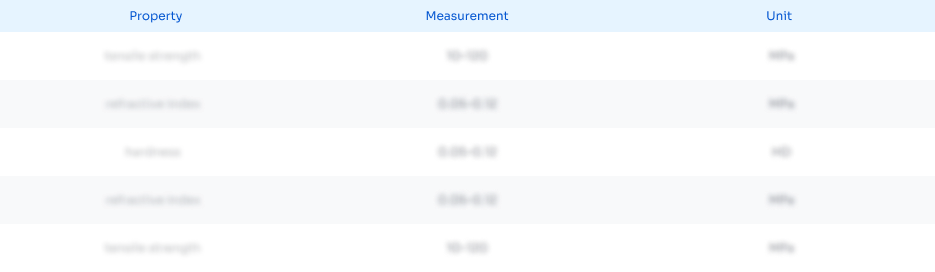
Abstract
Description
Claims
Application Information
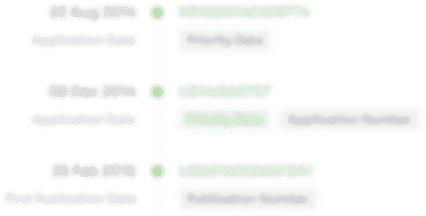
- R&D Engineer
- R&D Manager
- IP Professional
- Industry Leading Data Capabilities
- Powerful AI technology
- Patent DNA Extraction
Browse by: Latest US Patents, China's latest patents, Technical Efficacy Thesaurus, Application Domain, Technology Topic, Popular Technical Reports.
© 2024 PatSnap. All rights reserved.Legal|Privacy policy|Modern Slavery Act Transparency Statement|Sitemap|About US| Contact US: help@patsnap.com