Deep learning differential privacy protection method
A differential privacy and deep learning technology, applied in the field of information system security, can solve problems such as parameter shocks, and achieve the effects of improving usability, improving practical significance, and high data availability
- Summary
- Abstract
- Description
- Claims
- Application Information
AI Technical Summary
Problems solved by technology
Method used
Image
Examples
Embodiment 1
[0051] to combine figure 1 , a deep learning differential privacy protection method of the present embodiment, the steps of which are:
[0052] Step 1. Construct a convolutional neural network with two convolutional layers and three fully connected layers, introduce differential privacy theory into network parameter optimization, and add Gaussian noise that satisfies the Gaussian mechanism. The specific process is as follows:
[0053] Initialize, establish a convolutional neural network with two convolutional layers and three fully connected layers, and initialize the model parameters of the convolutional neural network, such as image 3 shown. Introduce (ε,δ)-differential privacy as shown in formula (1):
[0054] Pr[M(D)∈S M ]≤e ε ×Pr[M(D’)∈S M ]+δ (1)
[0055] Among them, M is a given random algorithm; D and D' are neighbor data sets with a difference of at most one record; S M are all possible outputs of random algorithm M on data sets D and D'. The degree of privac...
PUM
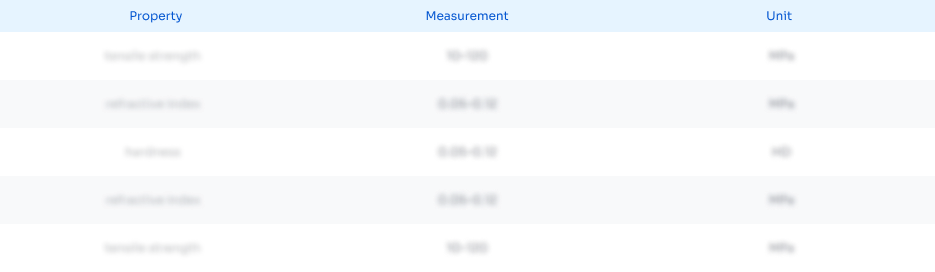
Abstract
Description
Claims
Application Information
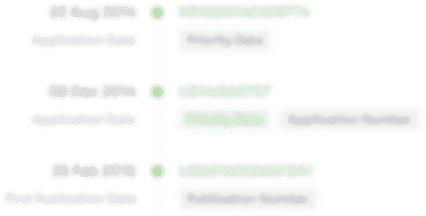
- R&D Engineer
- R&D Manager
- IP Professional
- Industry Leading Data Capabilities
- Powerful AI technology
- Patent DNA Extraction
Browse by: Latest US Patents, China's latest patents, Technical Efficacy Thesaurus, Application Domain, Technology Topic, Popular Technical Reports.
© 2024 PatSnap. All rights reserved.Legal|Privacy policy|Modern Slavery Act Transparency Statement|Sitemap|About US| Contact US: help@patsnap.com