Face activity unit detection method based on multi-task learning
A multi-task learning and detection method technology, applied in the field of facial activity unit detection based on multi-task learning
- Summary
- Abstract
- Description
- Claims
- Application Information
AI Technical Summary
Problems solved by technology
Method used
Image
Examples
Embodiment Construction
[0076] In order to make the technical problems, technical solutions and advantages to be solved by the present invention clearer, the following will describe in detail with reference to the drawings and specific embodiments.
[0077] Embodiments of the present invention provide a method for detecting facial activity units based on multi-task learning, such as figure 1 As shown, the method includes the following steps:
[0078] Auxiliary task learning: the AlexNet network before the global average pooling layer is used as a shared structure to extract shared global facial features, and the extracted shared global facial features are sent to independent network structures related to tasks to obtain the output of auxiliary tasks; The output of the auxiliary tasks includes the output of landmark detection, gender recognition, head pose estimation and expression recognition;
[0079] Main task learning: Cut the face into the upper half face and the lower half face, and input them ...
PUM
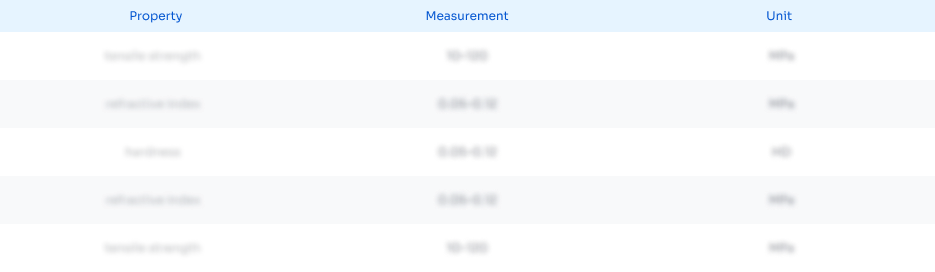
Abstract
Description
Claims
Application Information
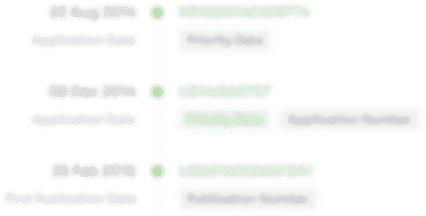
- R&D
- Intellectual Property
- Life Sciences
- Materials
- Tech Scout
- Unparalleled Data Quality
- Higher Quality Content
- 60% Fewer Hallucinations
Browse by: Latest US Patents, China's latest patents, Technical Efficacy Thesaurus, Application Domain, Technology Topic, Popular Technical Reports.
© 2025 PatSnap. All rights reserved.Legal|Privacy policy|Modern Slavery Act Transparency Statement|Sitemap|About US| Contact US: help@patsnap.com