Method of using generative adversarial network in image restoration
An image and network technology, applied in the field of computer vision, can solve the problems of mild boundary artifacts, inconsistent surrounding areas, blurred texture structure of images, etc., to achieve the effect of improving repair accuracy, good repair effect, and improved visual effect
- Summary
- Abstract
- Description
- Claims
- Application Information
AI Technical Summary
Problems solved by technology
Method used
Image
Examples
Embodiment
[0067] The present invention analyzes and compares the method proposed by the present invention (hereinafter referred to as PRGAN) and three kinds of deep learning repair methods proposed in the past three years, and CE represents the method proposed by Pathak et al. in the experimental results [16] , GL means the method proposed by Iizuka et al. [5] , PConv represents the method proposed by Liu et al. [8] . Both CE and GL are representative works in the field of image inpainting with regular masks, while PConv can represent the frontier method in image inpainting with irregular masks. PConv-GAN represents the image restoration network module in the method proposed by the present invention, and Res-GAN represents the image optimization network module in the method of the present invention.
[0068] Figure 5 and Figure 6 Shown is the method PRGAN proposed by the present invention and three advanced methods: CE [16] , GL [5] and PConv [8] Qualitative comparison results ...
PUM
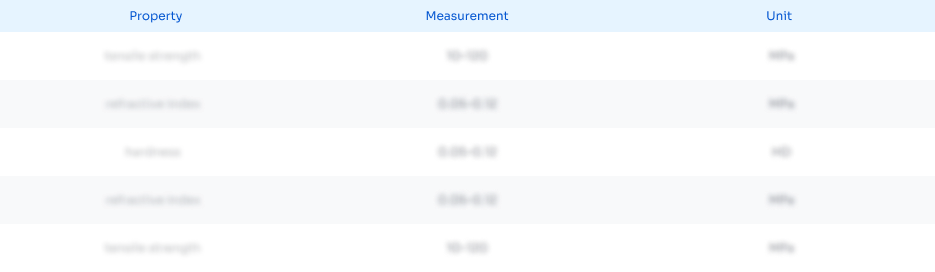
Abstract
Description
Claims
Application Information
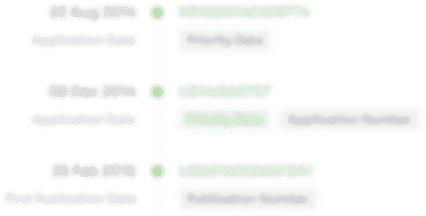
- R&D Engineer
- R&D Manager
- IP Professional
- Industry Leading Data Capabilities
- Powerful AI technology
- Patent DNA Extraction
Browse by: Latest US Patents, China's latest patents, Technical Efficacy Thesaurus, Application Domain, Technology Topic.
© 2024 PatSnap. All rights reserved.Legal|Privacy policy|Modern Slavery Act Transparency Statement|Sitemap