Cross-layer multi-model feature fusion and convolutional decoding-based image description method
A technology of feature fusion and image description, applied in neural learning methods, biological neural network models, still image data retrieval, etc., can solve problems such as inaccurate information description
- Summary
- Abstract
- Description
- Claims
- Application Information
AI Technical Summary
Problems solved by technology
Method used
Image
Examples
Embodiment 1
[0028] Such as Figure 1-5 The illustrated embodiment of the present invention provides a cross-layer multi-model feature fusion and image description method based on convolutional decoding, including the following steps:
[0029] S1. Firstly, in the vision module, the low-level and high-level cross-layer image feature fusion is realized in a single model, and then the feature maps obtained by multiple visual feature extraction models are averagely fused, and each sentence contained in the corresponding image is combined. words are mapped to words with D e dimensional embedding space, get their embedding vector sequences, and then obtain the final text features through 6 layers of causal convolution operations. When performing visual feature extraction, the rich feature information has a good guiding effect on the image description results, so using three A VGG16 structure is used as the extraction module of image visual features. At the same time, in order to fuse low-level ...
Embodiment 2
[0046] Such as Figure 1-7 The shown embodiment of the present invention provides a cross-layer multi-model feature fusion and image description method based on convolutional decoding, using VGG-16 and language-CNN (that is, the language module used in the present invention) to train the model, and its As the benchmark model CNN+CNN (Baseline), then on the basis of Baseline, add multiple VGG-16 networks, and realize cross-layer feature fusion in each VGG-16, and use the trained benchmark model parameters to carry out the model Initialization, retraining, on the MSCOCO dataset, some experimental results are as follows:
[0047] R1: A hamburger and a salad sitting on top of a table.
[0048] R2: A salad and a sandwich wait to be eaten at a restaurant. R3: An outside dining area with tables and chairs highlighting a salad and sandwich.
[0049] R4: A sandwich and a salad are on a tray on a wooden table.
[0050] R5: A table with a bowl of food, sandwich and wine glass sitting ...
PUM
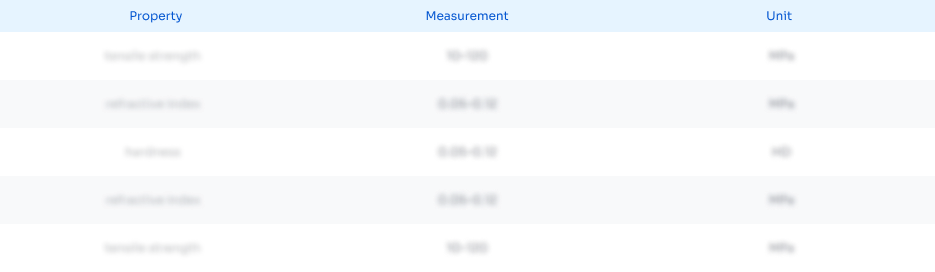
Abstract
Description
Claims
Application Information
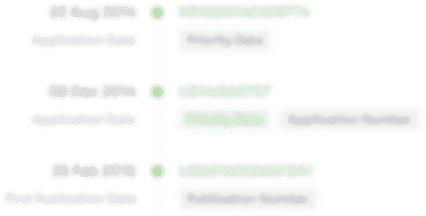
- R&D
- Intellectual Property
- Life Sciences
- Materials
- Tech Scout
- Unparalleled Data Quality
- Higher Quality Content
- 60% Fewer Hallucinations
Browse by: Latest US Patents, China's latest patents, Technical Efficacy Thesaurus, Application Domain, Technology Topic, Popular Technical Reports.
© 2025 PatSnap. All rights reserved.Legal|Privacy policy|Modern Slavery Act Transparency Statement|Sitemap|About US| Contact US: help@patsnap.com