Multi-modal emotion analysis method and system based on deep learning for acupuncture
A emotion analysis and deep learning technology, applied in the field of emotion recognition, can solve the problems of not considering the influence of feature weights, fixation of different feature weights, EEG, heart rate interference, etc., to achieve the effect of weakening the interference of noise
- Summary
- Abstract
- Description
- Claims
- Application Information
AI Technical Summary
Problems solved by technology
Method used
Image
Examples
Embodiment 1
[0170] According to a specific embodiment of the present invention, the present invention provides a deep learning-based multimodal emotion analysis method for acupuncture. The present invention will be described through a set of experiments below. In this experiment, 18 times of acupuncture treatments were selected. Face screenshots and EEG signal interception data segments are used for experiments, including the following steps:
[0171] Step 1: Collect facial expression images of patients during acupuncture, process the collected facial expression images, and extract Gabor wavelet features of facial expressions through Gabor wavelet transform;
[0172] Process the patient’s facial expression images at the same time corresponding to the 18-time decision curve library, reduce each picture to a uniform size, and obtain the historical facial expression image library, as shown in the attached Figure 5 shown.
[0173] Extract texture features from the pictures in the above-ment...
Embodiment 2
[0193] The present invention adopts a multi-modal feature fusion method, and uses variable weight sparse linear fusion to perform weighting processing on the extracted features of different modal images, and synthesizes a feature vector. The feature fusion weighting formula is expressed as follows:
[0194] O(x)=γK(x)+(1-γ)F(x) (1)
[0195] Wherein: K(x) represents the feature of EEG curve image;
[0196] F(x) represents facial expression features;
[0197] γ is the empirical coefficient.
[0198] Table 2 shows the results of each single mode and the fusion of these two modes, with Figure 9 Accuracy comparison plots using different modalities are shown.
[0199] Table 2 Classification results of each mode and fusion
[0200]
[0201] From attached Figure 9 It can be seen from the figure that the effect of single-modal facial expression on emotion classification is better than that of EEG signal curve, and the accuracy of emotion classification can be improved through...
Embodiment 3
[0203] In order to further study the complementary features of facial expressions and EEG signal curves, this embodiment analyzes the confusion matrix of each modality, revealing the advantages and disadvantages of each modality.
[0204] attached Figure 10a , Figure 10b and Figure 10c (Each column in each figure represents predicted emotion classification information, and each row represents actual emotion classification information.) A confusion matrix based on facial expression and EEG signal curves is given. Figure 10a For the effect of facial expression on emotion classification, Figure 10b is the effect of EEG signals on emotion classification, Figure 10c The effect of mixing two modalities on emotion classification. The experimental results show that facial expressions and EEG curves have different discriminative powers for emotion recognition. Facial expressions are better for the classification of happy emotions, but both facial expressions and EEG signals a...
PUM
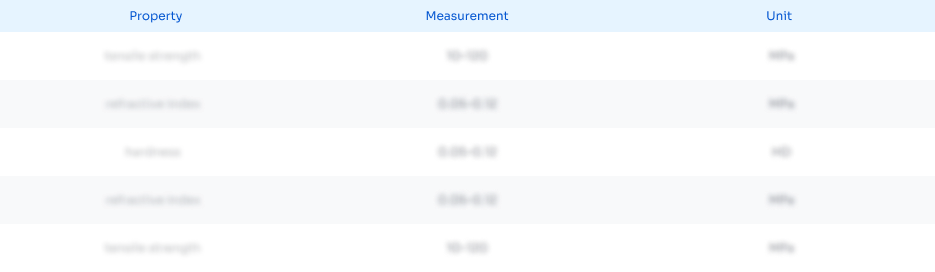
Abstract
Description
Claims
Application Information
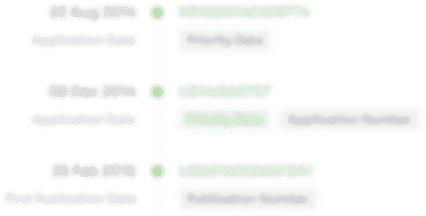
- R&D
- Intellectual Property
- Life Sciences
- Materials
- Tech Scout
- Unparalleled Data Quality
- Higher Quality Content
- 60% Fewer Hallucinations
Browse by: Latest US Patents, China's latest patents, Technical Efficacy Thesaurus, Application Domain, Technology Topic, Popular Technical Reports.
© 2025 PatSnap. All rights reserved.Legal|Privacy policy|Modern Slavery Act Transparency Statement|Sitemap|About US| Contact US: help@patsnap.com