Short-term power load prediction method based on dimensionality reduction and improved neural network
A short-term power load, neural network technology, applied in neural learning methods, biological neural network models, forecasting and other directions, can solve the problems of poor ELM stability, increased power operating costs, and low prediction accuracy, and improve short-term power load. Predict the process, improve accuracy, reduce the effect of calculated data
- Summary
- Abstract
- Description
- Claims
- Application Information
AI Technical Summary
Problems solved by technology
Method used
Image
Examples
Embodiment
[0083] The SNE-MFOELM prediction model is established based on the electric load data and meteorological data of a certain place in the United States, and the daily electric load of this place is predicted, and the load data and seven meteorological influencing factors of this place from January 1 to 8, 2006 are selected. (Wind speed L1, air pressure L2, precipitation L3, temperature L4, cloud cover L5, humidity L6, SO2 concentration L7), sampling every 30 minutes, 24 hours a day, a total of 48 sampling points, as research data. The dimensionality reduction meteorological sequence and load sequence from January 1st to 3rd are used as the input of the training set, and the load sequence on the 4th is used as the output of the training set; the dimensionality reduction meteorological sequence and load sequence from January 5th to 7th are used as the test set Input, 8 loads as the output of the test set.
[0084] The forecasting process follows figure 1 The flow chart proceeds. ...
PUM
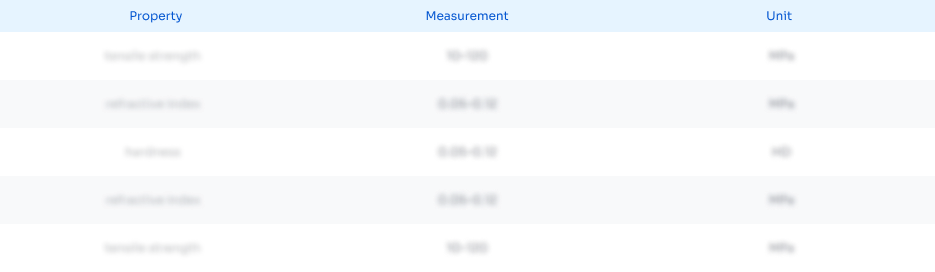
Abstract
Description
Claims
Application Information
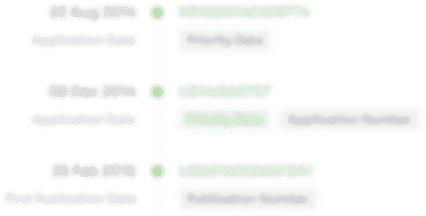
- R&D Engineer
- R&D Manager
- IP Professional
- Industry Leading Data Capabilities
- Powerful AI technology
- Patent DNA Extraction
Browse by: Latest US Patents, China's latest patents, Technical Efficacy Thesaurus, Application Domain, Technology Topic.
© 2024 PatSnap. All rights reserved.Legal|Privacy policy|Modern Slavery Act Transparency Statement|Sitemap