Remote sensing image road segmentation method based on convolutional neural network weak supervised learning
A technology of convolutional neural network and remote sensing image, which is applied in the field of remote sensing image road segmentation based on convolutional neural network weak supervision learning, can solve problems such as unfavorable production and application, irregular road edges, and increase the amount of calculation, and achieve algorithm efficiency and The effect of performance improvement and labeling cost reduction
- Summary
- Abstract
- Description
- Claims
- Application Information
AI Technical Summary
Problems solved by technology
Method used
Image
Examples
Embodiment Construction
[0030] The specific implementation manner of the present invention is illustrated below through examples and accompanying drawings.
[0031]An embodiment of the present invention provides a remote sensing image road segmentation method based on convolutional neural network weak supervision learning, such as figure 1 The total shown is divided into label propagation, construction, training and prediction of the convolutional neural network model of the dual-branch encoder-decoder structure.
[0032] Firstly, a sample library is constructed based on the existing remote sensing images and the corresponding road centerline vector files. Multiple remote sensing images are spliced to obtain a complete image, and the images are resampled and cropped to obtain images with appropriate resolution and road coverage. The pixels corresponding to the road centerline in vector format are marked as roads to obtain rasterized road centerline data. During the format conversion process, it i...
PUM
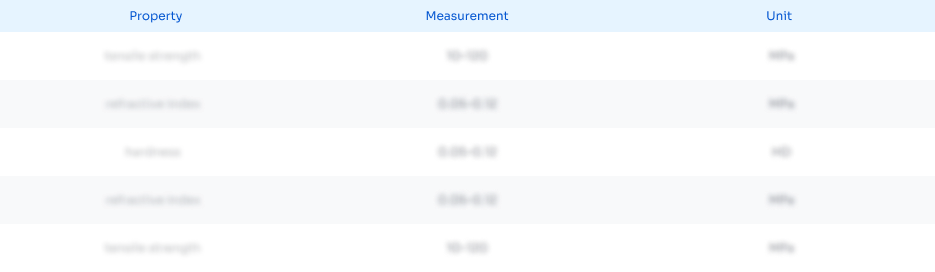
Abstract
Description
Claims
Application Information
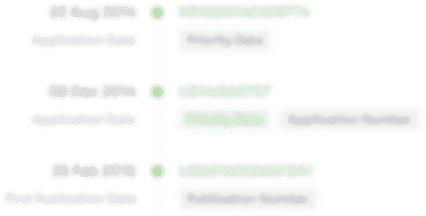
- R&D Engineer
- R&D Manager
- IP Professional
- Industry Leading Data Capabilities
- Powerful AI technology
- Patent DNA Extraction
Browse by: Latest US Patents, China's latest patents, Technical Efficacy Thesaurus, Application Domain, Technology Topic, Popular Technical Reports.
© 2024 PatSnap. All rights reserved.Legal|Privacy policy|Modern Slavery Act Transparency Statement|Sitemap|About US| Contact US: help@patsnap.com