Retinal fundus vessel segmentation method based on deep multi-scale attention convolutional neural network
A convolutional neural network and attention technology, applied in retinal vessel segmentation, computer technology and pattern recognition, to achieve clear segmentation probability map, accurate background segmentation, and avoid feature loss
- Summary
- Abstract
- Description
- Claims
- Application Information
AI Technical Summary
Problems solved by technology
Method used
Image
Examples
Embodiment Construction
[0023] According to one embodiment of the present invention, a retinal fundus vessel segmentation method based on a deep multi-scale attentional convolutional neural network is proposed. A dual attention mechanism is introduced in the connection path between the encoder and the decoder of the U-Net architecture, and a multi-scale feature fusion module is introduced at the output of each layer of the decoder to finally obtain the retinal fundus blood vessel segmentation result.
[0024] Below in conjunction with the accompanying drawings, the specific implementation of a retinal fundus blood vessel segmentation method based on a deep multi-scale attention convolutional neural network proposed by the present invention will be described in detail:
[0025] Step 1: Obtain DRIVE, an internationally publicized color retinal fundus blood vessel dataset;
[0026] Step 2: Select the pictures used for training in the data set, adjust their size to 512×512 pixels, design a random data en...
PUM
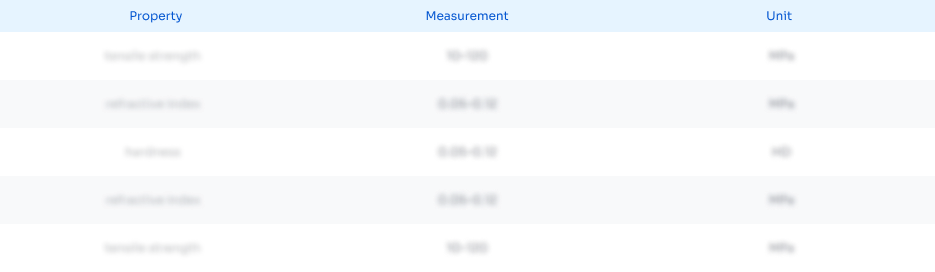
Abstract
Description
Claims
Application Information
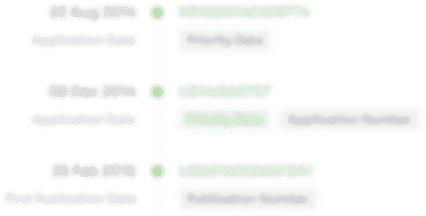
- R&D Engineer
- R&D Manager
- IP Professional
- Industry Leading Data Capabilities
- Powerful AI technology
- Patent DNA Extraction
Browse by: Latest US Patents, China's latest patents, Technical Efficacy Thesaurus, Application Domain, Technology Topic, Popular Technical Reports.
© 2024 PatSnap. All rights reserved.Legal|Privacy policy|Modern Slavery Act Transparency Statement|Sitemap|About US| Contact US: help@patsnap.com