Monitoring video target real-time query method based on edge cloud convolutional neural network cascading
A convolutional neural network and surveillance video technology, which is applied in the field of real-time query of surveillance video targets, can solve problems such as large network bandwidth requirements and data transmission delay, difficulty in meeting new requirements for real-time performance, and inability to guarantee query accuracy, etc. The effect of uploading data to the cloud, reducing data transmission delay, and improving query accuracy
- Summary
- Abstract
- Description
- Claims
- Application Information
AI Technical Summary
Problems solved by technology
Method used
Image
Examples
Embodiment Construction
[0037] The present invention will be further described in detail below in conjunction with the accompanying drawings and embodiments, which are explanations of the present invention rather than limitations.
[0038] Such as figure 1 Shown is the process logic block diagram of the present invention, the monitoring video target real-time query method based on edge-cloud convolutional neural network cascading of the present invention, comprises the following steps:
[0039] Step 1: When a user-defined query command is received, the specific CNN (convolutional neural network) based on the specific training data set and the specified query target will be fine-tuned and trained in the cloud server, and then the specific CNN will be deployed to the edge server;
[0040] Step 2: The edge server uses the target detection algorithm based on the difference between frames to detect and extract the moving target in the surveillance video;
[0041] Step 3: The edge server uses the load-base...
PUM
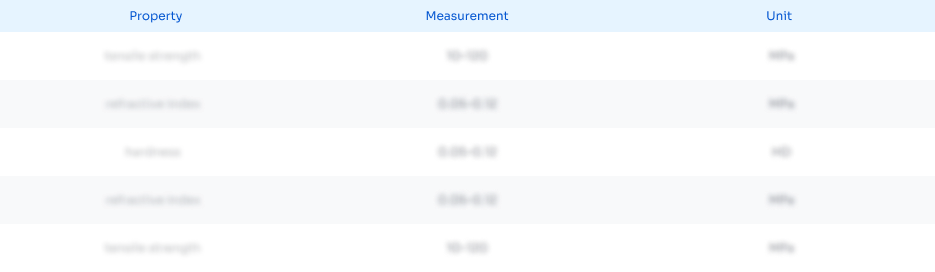
Abstract
Description
Claims
Application Information
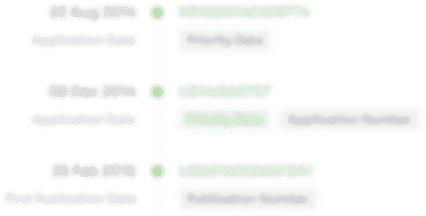
- Generate Ideas
- Intellectual Property
- Life Sciences
- Materials
- Tech Scout
- Unparalleled Data Quality
- Higher Quality Content
- 60% Fewer Hallucinations
Browse by: Latest US Patents, China's latest patents, Technical Efficacy Thesaurus, Application Domain, Technology Topic, Popular Technical Reports.
© 2025 PatSnap. All rights reserved.Legal|Privacy policy|Modern Slavery Act Transparency Statement|Sitemap|About US| Contact US: help@patsnap.com