Wafer CMP material removal rate prediction method of GMDH neural network
A neural network and prediction method technology, which is applied in the field of wafer CMP material removal rate prediction, can solve the problem that the material removal rate in CMP technology cannot be accurately obtained.
- Summary
- Abstract
- Description
- Claims
- Application Information
AI Technical Summary
Problems solved by technology
Method used
Image
Examples
Embodiment Construction
[0080] The present invention will be further described below in combination with specific embodiments. It should be understood that these examples are only used to illustrate the present invention and are not intended to limit the scope of the present invention. In addition, it should be understood that after reading the teachings of the present invention, those skilled in the art can make various changes or modifications to the present invention, and these equivalent forms also fall within the scope defined by the appended claims of the present application.
[0081] A method for predicting the material removal rate of wafer CMP by GMDH neural network, the flow chart of which is as follows Figure 1~2 As shown, it specifically includes the following steps:
[0082] (1) Obtain the polished sample data set after removing outliers; the number of samples is n, and each sample contains 25 process variables and corresponding MRR values (ie, material removal rate);
[0083] Proce...
PUM
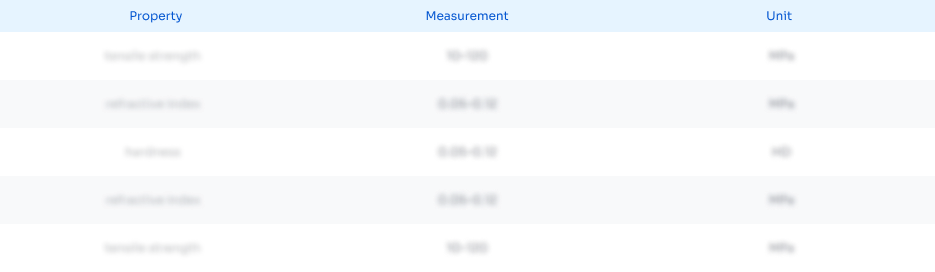
Abstract
Description
Claims
Application Information
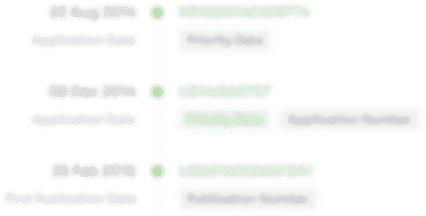
- R&D Engineer
- R&D Manager
- IP Professional
- Industry Leading Data Capabilities
- Powerful AI technology
- Patent DNA Extraction
Browse by: Latest US Patents, China's latest patents, Technical Efficacy Thesaurus, Application Domain, Technology Topic, Popular Technical Reports.
© 2024 PatSnap. All rights reserved.Legal|Privacy policy|Modern Slavery Act Transparency Statement|Sitemap|About US| Contact US: help@patsnap.com