MU-MISO hybrid precoding design method based on multi-agent deep reinforcement learning
A technology of reinforcement learning and design method, applied in the field of MU-MISO hybrid precoding design, which can solve the problems of high complexity of hybrid precoding and poor attainable rate performance
- Summary
- Abstract
- Description
- Claims
- Application Information
AI Technical Summary
Problems solved by technology
Method used
Image
Examples
Embodiment Construction
[0052] Below in conjunction with accompanying drawing, the present invention will be further described:
[0053] Such as figure 1 As shown, the MU-MISO hybrid precoding design method based on multi-agent deep reinforcement learning of the present invention considers a MU-MISO downlink, and the base station performs hybrid beamforming design according to the following steps:
[0054] Step 1. Base station configuration N t = 64 transmitting antennas, serving K = 8 single-antenna users; the base station knows the channel matrix h between it and each user k k ; Let t=0; Initialize Y=2 deep reinforcement learning agents composed of neural networks to learn the simulated precoding matrix F respectively RF,i And calculate the corresponding digital precoding matrix F D,i ; An evaluation network is used to coordinate the behavior of each agent; a reward value prediction network is used to accelerate the exploration of each agent; the evaluation network and reward value prediction ne...
PUM
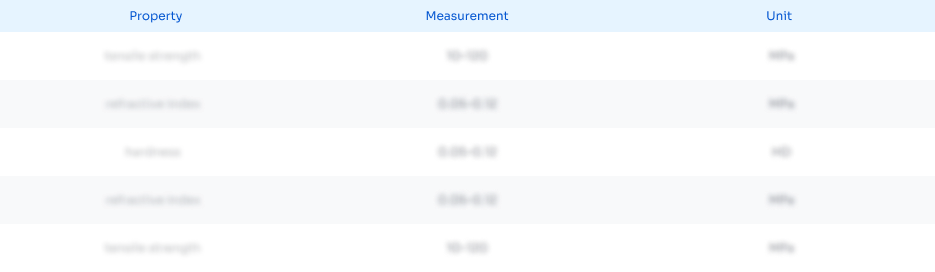
Abstract
Description
Claims
Application Information
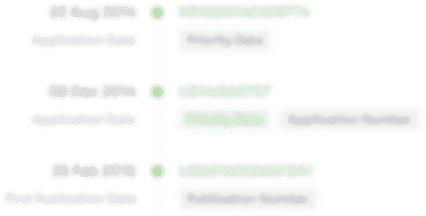
- R&D Engineer
- R&D Manager
- IP Professional
- Industry Leading Data Capabilities
- Powerful AI technology
- Patent DNA Extraction
Browse by: Latest US Patents, China's latest patents, Technical Efficacy Thesaurus, Application Domain, Technology Topic, Popular Technical Reports.
© 2024 PatSnap. All rights reserved.Legal|Privacy policy|Modern Slavery Act Transparency Statement|Sitemap|About US| Contact US: help@patsnap.com