CNN (Convolutional Neural Network)-based method for quickly identifying low-frequency oscillation modal characteristics of power system
A low-frequency oscillation and power system technology, applied in neural learning methods, character and pattern recognition, electrical components, etc., can solve problems such as insufficient consideration of the non-stationary characteristics of the measured oscillation signal
- Summary
- Abstract
- Description
- Claims
- Application Information
AI Technical Summary
Problems solved by technology
Method used
Image
Examples
Embodiment 1
[0119] In order to verify whether the algorithm of the present invention can identify the power system superimposing new oscillation modes in the oscillation process, the ideal LFO test signal is constructed as follows:
[0120]
[0121] In formula (9), ε(t) represents a step function, and η(t) represents a noise signal.
[0122] The LFO signal image as Figure 4 As shown, the signal length is 12s, and the signal-to-noise ratio SNR=10dB. Before 5s, the signal contains two modes, where the frequency f 1 = 0.84Hz, f 2 =1.21Hz, attenuation factor σ 1 =0.12, σ 2 =-0.15. When t=5s, a new oscillation mode is introduced, its frequency f 3 = 1.58Hz, attenuation factor σ 1 =0.3. After 6s, due to the σ in the initial mode 2 =-0.15 belongs to the strong attenuation component, and this mode is no longer counted as the dominant mode at this time, so the signal still contains two modes, where the frequency f 1 = 0.84Hz, f 3 = 1.58Hz, attenuation factor σ 1 =0.12, σ 2 = 0.3. ...
Embodiment 2
[0129] In order to verify the actual identification effect of the present invention, a piece of LFO measured data is obtained from the power system. Such as Figure 5 As shown in , this section of LFO signal is excited by two small disturbances, located at 4s and 7s respectively. In order to identify the LFO modal characteristics excited after the two disturbances, the data after the disturbance is intercepted as the LFO signal to be tested in this embodiment.
[0130] Step 7 acquires samples of the LFO signal to be tested through the sliding time window. The sliding window length is 4s, the sliding interval is 1s, and the sampling frequency is 100Hz. In order to verify the applicability of the present invention in the case of multiple disturbances in the system, it is necessary to use sliding window sampling after two disturbances occur. In this embodiment, two segments of signals are selected as identification objects, respectively 4.2-8.2s and 7.05-11.05s. Among them, 4...
PUM
Property | Measurement | Unit |
---|---|---|
Snr | aaaaa | aaaaa |
Abstract
Description
Claims
Application Information
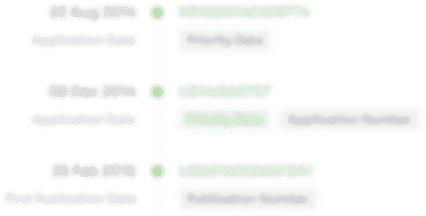
- R&D Engineer
- R&D Manager
- IP Professional
- Industry Leading Data Capabilities
- Powerful AI technology
- Patent DNA Extraction
Browse by: Latest US Patents, China's latest patents, Technical Efficacy Thesaurus, Application Domain, Technology Topic, Popular Technical Reports.
© 2024 PatSnap. All rights reserved.Legal|Privacy policy|Modern Slavery Act Transparency Statement|Sitemap|About US| Contact US: help@patsnap.com