Construction method of super-resolution convolutional neural network model
A convolutional neural network and convolutional neural network technology, applied in the field of super-resolution convolutional neural network model construction, can solve the problems of large amount of Tensor data, blurring, etc., and achieve reduced memory consumption, no obvious decline, and high speed Improved effect
- Summary
- Abstract
- Description
- Claims
- Application Information
AI Technical Summary
Problems solved by technology
Method used
Image
Examples
Embodiment 1
[0024] according to Figure 1-2 Shown, a kind of construction method of super-resolution convolutional neural network model, described construction method comprises the following steps,
[0025] Step 1: Build a convolutional neural network model;
[0026] Step 2: training the convolutional neural network model of step 1;
[0027] Step 3: Deploy the convolutional neural network model trained in step 2 to realize the image super-resolution function.
[0028] Further, the step 1 specifically includes constructing a convolutional neural network model using two methods of five residual blocks and sub-pixel convolution.
[0029] Further, the construction method of the sub-pixel convolution is specifically to convert the low-resolution feature map (640x360x27) with small length and width dimensions and deep channel depth into a large length and width dimension through pixel shuffling. , high-resolution feature maps (1920x1080x3) with shallow channel depths.
[0030] Further, the ...
Embodiment 2
[0036] Step 1: PSNR (Peak Signal-to-Noise Ratio) and SSIM (Structural Similarity) are evaluation indicators for evaluating the quality of neural network output pictures, the higher the better, and the fewer parameters and training time, the better.
[0037] Due to the use of sub-pixel convolution technology, the images flowing in the network are low-resolution images. The final effect is that the speed of the network inference stage is greatly improved; the memory consumption of the network inference stage is reduced; the final effect of the network is improved.
[0038] If the task is 4 times super-resolution, the size of the low-resolution image is 100*100, and the size of the original high-resolution image is 400*400. If sub-pixel convolution is not used, the network input is a picture pre-enlarged by the bicubic interpolation algorithm , that is, the size of 400*400, such as using sub-pixel convolution, the input does not need to be pre-amplified, that is, the input is 100*...
PUM
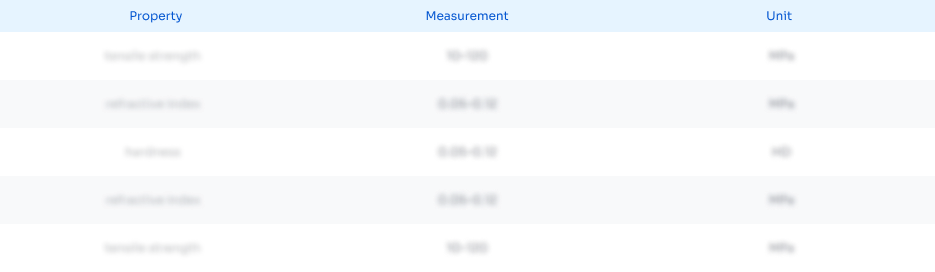
Abstract
Description
Claims
Application Information
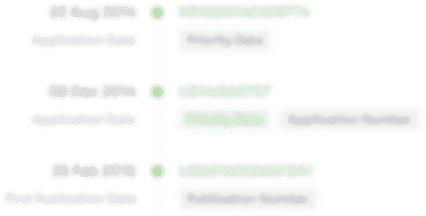
- R&D Engineer
- R&D Manager
- IP Professional
- Industry Leading Data Capabilities
- Powerful AI technology
- Patent DNA Extraction
Browse by: Latest US Patents, China's latest patents, Technical Efficacy Thesaurus, Application Domain, Technology Topic, Popular Technical Reports.
© 2024 PatSnap. All rights reserved.Legal|Privacy policy|Modern Slavery Act Transparency Statement|Sitemap|About US| Contact US: help@patsnap.com