Method for predicting residual cycle life of lithium battery in energy storage system based on Xgboost model
A technology for cycle life and model prediction, applied in the power field, can solve the problems of inability to disassemble lithium batteries, separate testing, and a large number of other problems, avoiding overfitting and underfitting, speeding up training, and large data samples. Effect
- Summary
- Abstract
- Description
- Claims
- Application Information
AI Technical Summary
Problems solved by technology
Method used
Image
Examples
Embodiment 1
[0044] refer to figure 1 , a method for predicting the remaining cycle life of a lithium battery in an energy storage system based on the Xgboost model, comprising the following steps:
[0045] S1: Data collection, collecting the actual operation data of the lithium battery during the operation of the energy storage power station, including battery cluster voltage, battery cluster temperature, battery cluster current, battery cluster accumulative charge and discharge times and other available data;
[0046] S2: Data cleaning, organize the collected data, remove invalid, abnormal, and partially missing data, and retain valid data;
[0047] S3: Feature selection, constructing feature data by calculating the original data, analyzing the correlation between feature data, making trade-offs, and selecting appropriate feature data as the input and output of the model;
[0048] S4: Model selection, choose the Xgboost model to predict the remaining cycle life of lithium batteries, usi...
Embodiment 2
[0092] refer to figure 1 , a method for predicting the remaining cycle life of a lithium battery in an energy storage system based on the Xgboost model, comprising the following steps:
[0093] S1: Data collection, collecting the actual operation data of the lithium battery during the operation of the energy storage power station, including battery cluster voltage, battery cluster temperature, battery cluster current, battery cluster accumulative charge and discharge times and other available data;
[0094] S2: Data cleaning, organize the collected data, remove invalid, abnormal, and partially missing data, and retain valid data;
[0095] S3: Feature selection, constructing feature data by calculating the original data, analyzing the correlation between feature data, making trade-offs, and selecting appropriate feature data as the input and output of the model;
[0096] S4: Model selection, choose the Xgboost model to predict the remaining cycle life of lithium batteries, usi...
PUM
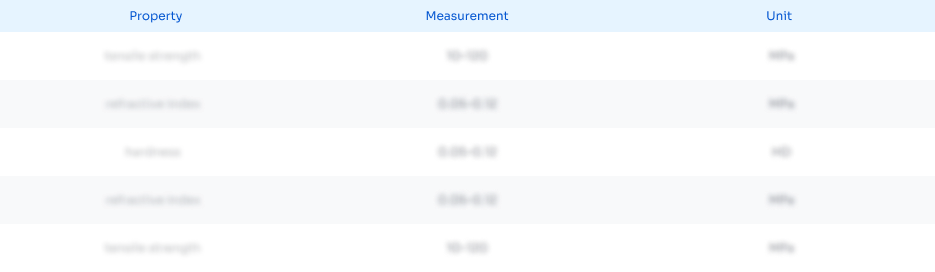
Abstract
Description
Claims
Application Information
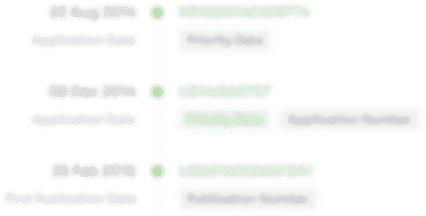
- R&D Engineer
- R&D Manager
- IP Professional
- Industry Leading Data Capabilities
- Powerful AI technology
- Patent DNA Extraction
Browse by: Latest US Patents, China's latest patents, Technical Efficacy Thesaurus, Application Domain, Technology Topic.
© 2024 PatSnap. All rights reserved.Legal|Privacy policy|Modern Slavery Act Transparency Statement|Sitemap