Aluminum Surface Defect Detection Method Based on Improved Cascade R-CNN
An improved technology for defect detection, applied in neural learning methods, biological neural network models, image enhancement, etc., can solve problems such as difficult detection of extreme aspect ratios, high false detection rate, etc., to alleviate the loss of precision and improve the detection rate , the effect of training step improvement
- Summary
- Abstract
- Description
- Claims
- Application Information
AI Technical Summary
Problems solved by technology
Method used
Image
Examples
Embodiment Construction
[0039] The present invention will be further described below in conjunction with specific examples.
[0040] The example uses real collected aluminum surface picture data, including 10 kinds of defects such as non-conductivity, scratches, orange peel, and dirty spots, several normal pictures and marked defect pictures, and the picture size is 2560*1920.
[0041] like figure 1 and figure 2 As shown, the improved Cascade R-CNN-based aluminum surface defect detection method provided in this embodiment includes the following steps:
[0042] 1) Standardize the size of the aluminum surface picture set, and scale all the picture sizes to 1280*960. The pictures containing defects have corresponding defect labeling files, and the picture set is divided into a normal picture set and a defect picture set according to whether the picture contains a defect labeling file.
[0043] The aluminum surface picture set includes a defect picture set X containing defects, a normal picture set Y...
PUM
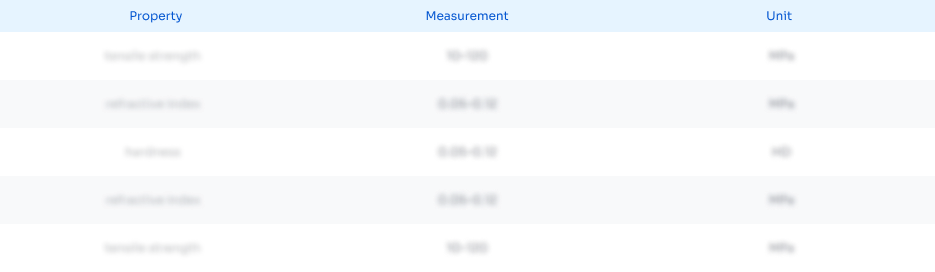
Abstract
Description
Claims
Application Information
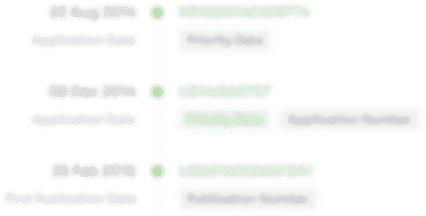
- R&D
- Intellectual Property
- Life Sciences
- Materials
- Tech Scout
- Unparalleled Data Quality
- Higher Quality Content
- 60% Fewer Hallucinations
Browse by: Latest US Patents, China's latest patents, Technical Efficacy Thesaurus, Application Domain, Technology Topic, Popular Technical Reports.
© 2025 PatSnap. All rights reserved.Legal|Privacy policy|Modern Slavery Act Transparency Statement|Sitemap|About US| Contact US: help@patsnap.com