Laser point cloud super-resolution reconstruction method based on self-attention generative adversarial network
A technology of super-resolution reconstruction and laser point cloud, which is applied in the field of super-resolution of laser point cloud data, can solve problems such as uneasy self-adaptation, unstructured and irregular point cloud data, and improve feature extraction efficiency and reduce Network runtime, beneficial effect of light weight
- Summary
- Abstract
- Description
- Claims
- Application Information
AI Technical Summary
Problems solved by technology
Method used
Image
Examples
Embodiment Construction
[0028] The present invention will be described in detail below in conjunction with the accompanying drawings and specific embodiments.
[0029] An embodiment of the present invention provides a laser point cloud super-resolution reconstruction method based on a self-attention generative confrontation network, such as figure 1 As shown, the method is specifically implemented through the following steps:
[0030] Step 101: Use the feature extraction module of the generator network to perform point cloud deep feature extraction on the input unordered sparse point cloud set containing N points.
[0031] Specifically, the laser point cloud feature F(N×C) is extracted from the sparse point cloud input P of size N×d, where d is the dimension of the point cloud, that is, the spatial coordinates, distance, reflection intensity, etc., d= 3 That is, only consider the spatial three-dimensional coordinate information, and input the sparse point cloud of N×d into the feature extraction uni...
PUM
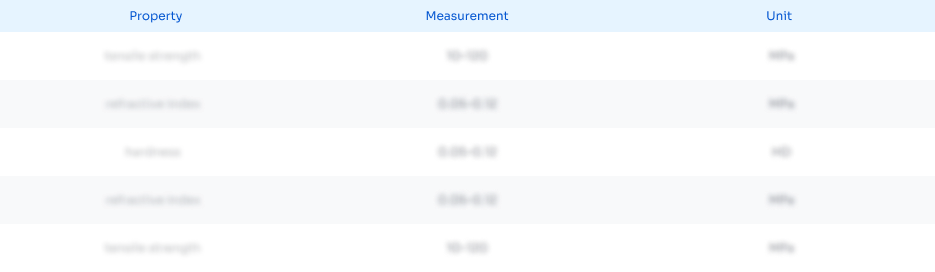
Abstract
Description
Claims
Application Information
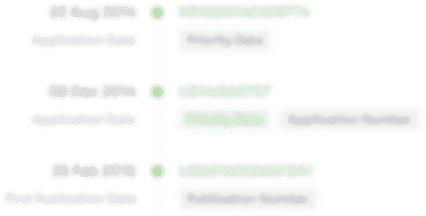
- R&D
- Intellectual Property
- Life Sciences
- Materials
- Tech Scout
- Unparalleled Data Quality
- Higher Quality Content
- 60% Fewer Hallucinations
Browse by: Latest US Patents, China's latest patents, Technical Efficacy Thesaurus, Application Domain, Technology Topic, Popular Technical Reports.
© 2025 PatSnap. All rights reserved.Legal|Privacy policy|Modern Slavery Act Transparency Statement|Sitemap|About US| Contact US: help@patsnap.com