Microgrid fault diagnosis method for optimizing extreme learning machine based on whale algorithm
An extreme learning machine and fault diagnosis technology, applied in neural learning methods, fault locations, machine learning and other directions, can solve problems such as difficulty in achieving optimal network performance, and achieve strong global optimization ability, fast convergence speed, and improved recognition accuracy. Effect
- Summary
- Abstract
- Description
- Claims
- Application Information
AI Technical Summary
Problems solved by technology
Method used
Image
Examples
Embodiment Construction
[0045] The present invention is achieved like this:
[0046] S1. According to the structure diagram of the micro-grid system, a simulation model of the grid-connected operation of the micro-grid including wind and storage is established, and each type of fault is simulated to obtain a three-phase fault voltage signal;
[0047] S2. Using wavelet packet analysis to decompose and reconstruct the collected three-phase fault voltage signal with three layers of wavelet packets, a total of 24 wavelet packet reconstruction signals are obtained, and the wavelet packet energy entropy structure of these 24 wavelet packet reconstruction signals is calculated Form a set of feature vectors as the input of the neural network;
[0048] S3. Use the whale optimization algorithm to optimize the input weight and threshold of the extreme learning machine to improve the global search ability of the network and make the network have better recognition accuracy;
[0049] S4. Finally, verify the effe...
PUM
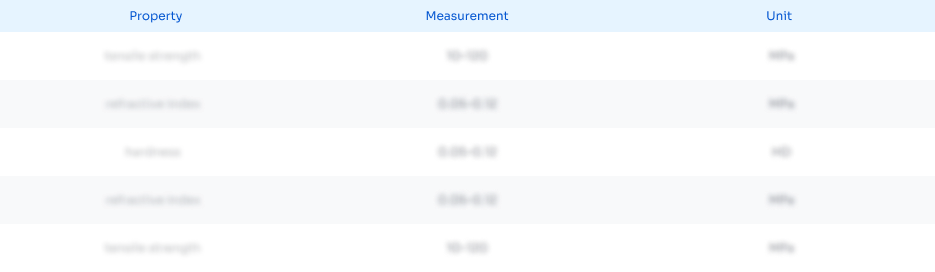
Abstract
Description
Claims
Application Information
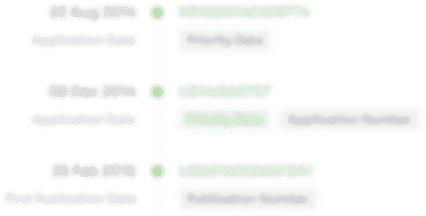
- R&D Engineer
- R&D Manager
- IP Professional
- Industry Leading Data Capabilities
- Powerful AI technology
- Patent DNA Extraction
Browse by: Latest US Patents, China's latest patents, Technical Efficacy Thesaurus, Application Domain, Technology Topic, Popular Technical Reports.
© 2024 PatSnap. All rights reserved.Legal|Privacy policy|Modern Slavery Act Transparency Statement|Sitemap|About US| Contact US: help@patsnap.com