Indoor scene semantic segmentation method based on improved full convolutional neural network
A convolutional neural network, indoor scene technology, applied in the field of indoor scene semantic segmentation based on improved full convolutional neural network, can solve the problems of insufficient feature expression extraction and large amount of parameters.
- Summary
- Abstract
- Description
- Claims
- Application Information
AI Technical Summary
Problems solved by technology
Method used
Image
Examples
Embodiment Construction
[0053] The present invention will be described in further detail below in conjunction with the accompanying drawings and specific embodiments.
[0054] An indoor scene semantic segmentation method based on the improved fully convolutional neural network proposed by the present invention, its overall realization block diagram is as follows figure 1 As shown, it includes two processes of training phase and testing phase;
[0055] The specific steps of the described training phase process are:
[0056] Step 1_1: Select Q pairs of original indoor scene RGB color images and Depth depth map images and real semantic segmentation images corresponding to each pair of original indoor scene images, and form a training set, and use the qth pair of original indoor scene images in the training set denoted as {RGB q (i,j),Depth q (i,j)}, the training set {RGB q (i,j),Depth q (i, j)} and the corresponding real semantic segmentation image are denoted as Then use the existing one-hot enc...
PUM
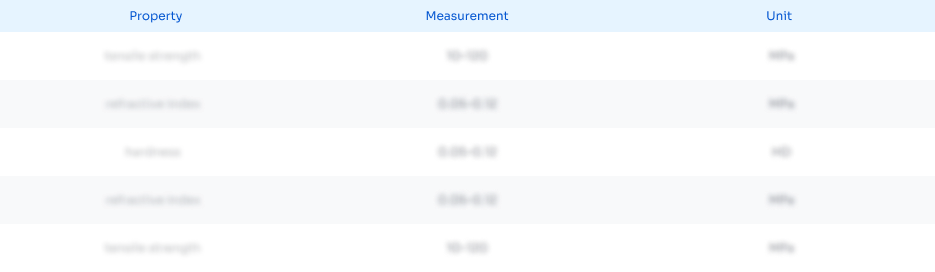
Abstract
Description
Claims
Application Information
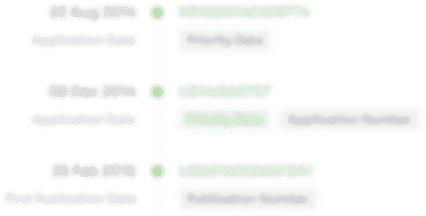
- R&D Engineer
- R&D Manager
- IP Professional
- Industry Leading Data Capabilities
- Powerful AI technology
- Patent DNA Extraction
Browse by: Latest US Patents, China's latest patents, Technical Efficacy Thesaurus, Application Domain, Technology Topic, Popular Technical Reports.
© 2024 PatSnap. All rights reserved.Legal|Privacy policy|Modern Slavery Act Transparency Statement|Sitemap|About US| Contact US: help@patsnap.com