Medical question-answering system construction method based on language model and entity matching
A technology of question answering system and construction method, applied in medical reference, semantic tool creation, health care informatics, etc., can solve problems such as low reliability, what food you can’t eat, and can’t be solved effectively
- Summary
- Abstract
- Description
- Claims
- Application Information
AI Technical Summary
Problems solved by technology
Method used
Image
Examples
Embodiment 1
[0028] A construction method of medical question answering system based on language model and entity matching, including S1. data collection, S2. deep neural network model design, S3. training named entity recognition model and constructing knowledge map, S4. constructing complete medical retrieval question answering system.
[0029] The data collected included web medical discussion posts and web public datasets from specific websites. Online medical discussion posts include medical questions actually asked by users and answers answered by professional doctors. The collected online medical discussion posts are cleaned and stored in ElasticSearch as a search data set. The network public datasets include medical natural language processing competition datasets and open datasets of open source websites, use the open source data of medical natural language processing competition datasets to train medical-related named entity recognition models; collect public datasets from open sou...
Embodiment 2
[0031] The difference between this embodiment and Embodiment 1 is that the design of the deep neural network model is based on the BERT model, and the twin network structure is introduced to form a deep neural network model structure combining the two, which is used for text matching or The task of answer selection.
[0032] Originally using the BERT model to do text matching tasks, you only need to connect two texts with [SEP] tags, input them into the BERT model, and then encode the CLS tags to output the final probability. The deep neural network model uses the BERT model to do text matching tasks. On the basis of keeping the original CLS label features unchanged, the question and answer texts are encoded in a single text, and then the Token Mean Vector is used as the feature of the sentence, and the three These features are spliced together by means of a twin network, and then input into a fully connected network for encoding. Being able to learn more similarities betwe...
Embodiment 3
[0034] The difference between this embodiment and Embodiment 1 is: training the named entity recognition model and constructing the knowledge map, and adding the entity matching step in the process of the traditional retrieval question answering system to increase the key entity's contribution to the entire retrieval process The role of entity matching uses two major aspects: named entity matching and knowledge graph entity matching. Specific steps include:
[0035] S3.1. Train the named entity recognition model, use the BERT-BiLSTM-CRF model for entity recognition, use the BERT model to obtain the features of the text, do not update the weight of the BERT model during training, only update the weight of the subsequent BiLSTM-CRF . The network public data set adopts the ccks 2019 Chinese medical entity recognition data set, which contains 6 important entity categories: disease, imaging examination, drug, chemical examination, operation and anatomical part. Training on this d...
PUM
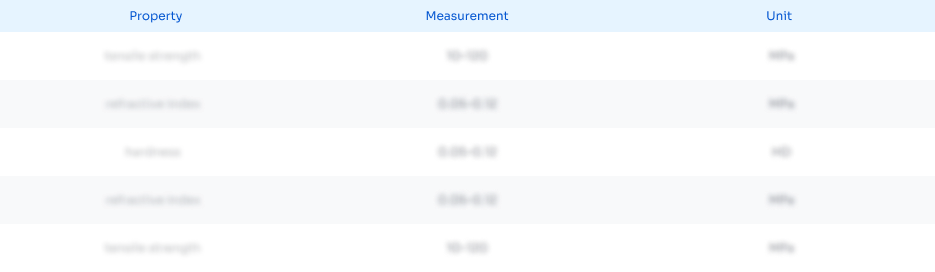
Abstract
Description
Claims
Application Information
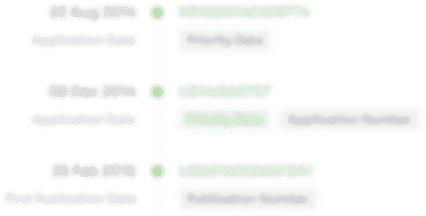
- R&D Engineer
- R&D Manager
- IP Professional
- Industry Leading Data Capabilities
- Powerful AI technology
- Patent DNA Extraction
Browse by: Latest US Patents, China's latest patents, Technical Efficacy Thesaurus, Application Domain, Technology Topic, Popular Technical Reports.
© 2024 PatSnap. All rights reserved.Legal|Privacy policy|Modern Slavery Act Transparency Statement|Sitemap|About US| Contact US: help@patsnap.com