Semi-supervised self-learning driven medical text disease identification method
An identification method and a semi-supervised technology, applied in the field of medical text disease identification, can solve problems such as insufficient information availability, poor understanding, and poor results
- Summary
- Abstract
- Description
- Claims
- Application Information
AI Technical Summary
Problems solved by technology
Method used
Image
Examples
Embodiment Construction
[0099] The technical solution of the semi-supervised self-learning-driven medical text disease recognition method provided by the present invention will be further described below in conjunction with the accompanying drawings, so that those skilled in the art can better understand the present invention and implement it.
[0100] Medical texts contain a large amount of medical knowledge. Using these clinical medical text data can assist in the prevention and diagnosis of diseases, and can also track the patient's diagnosis and treatment process, build a diagnosis and treatment cycle model, and construct a suitable diagnosis and treatment plan for patients. This has become a medical intelligence. important trends. At present, all medical texts contain unstructured text information, the most important of which are the patient's clinical information, patient history and diagnosis and treatment plan.
[0101] The present invention identifies the key features of the disease based on...
PUM
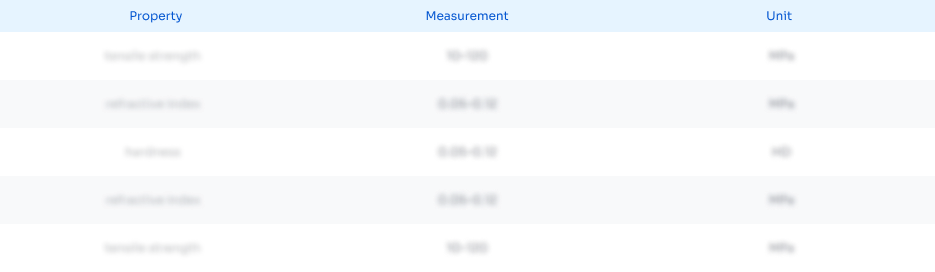
Abstract
Description
Claims
Application Information
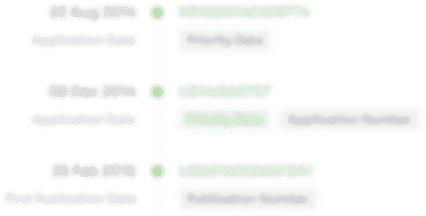
- R&D Engineer
- R&D Manager
- IP Professional
- Industry Leading Data Capabilities
- Powerful AI technology
- Patent DNA Extraction
Browse by: Latest US Patents, China's latest patents, Technical Efficacy Thesaurus, Application Domain, Technology Topic, Popular Technical Reports.
© 2024 PatSnap. All rights reserved.Legal|Privacy policy|Modern Slavery Act Transparency Statement|Sitemap|About US| Contact US: help@patsnap.com