Unsupervised depth representation learning method and system based on image translation
A technology of learning system and learning method, applied in the field of unsupervised deep representation learning method and system, can solve the problems of unsupervised prediction geometric transformation, prediction image cannot handle rotation invariant image, etc., and achieve the effect of excellent performance
- Summary
- Abstract
- Description
- Claims
- Application Information
AI Technical Summary
Problems solved by technology
Method used
Image
Examples
Embodiment 1
[0050] An unsupervised deep representation learning system based on image translation provided in this embodiment, such as figure 2 shown, including:
[0051] Image translation transformation module 11, for carrying out random translation transformation to image and generating auxiliary label;
[0052] Image masking module 12, is connected with image translation transformation module 11, is used for applying mask to the image after translation transformation;
[0053] The deep neural network 13 is connected with the image mask module 12, and is used to predict the actual auxiliary label of the image after applying the mask, and learn the depth representation of the image;
[0054] The regression loss function module 14 is connected with the deep neural network 13, and is used to update the parameters of the deep neural network based on the loss function;
[0055] The feature extraction module 15 is connected with the deep neural network 13 and is used to extract the represe...
Embodiment 2
[0080] An unsupervised deep representation learning system based on image translation provided in this embodiment is different from Embodiment 1 in that:
[0081] This embodiment is compared with existing methods on multiple data sets to verify the effectiveness of the above method.
[0082] data set:
[0083] CIFAR101: This dataset contains 60,000 color images of size 32×32, evenly distributed in 10 categories, that is, each category contains 6,000 images. Among them, 50,000 images are put into the training set, and the remaining 10,000 images are put into the test set.
[0084] CIFAR100: Similar to CIFAR10, it also contains 60,000 images, but is evenly distributed in 100 categories, each category contains 600 images. The number of samples in the training set and the test set is also 5:1.
[0085] STL10: Contains 13,000 labeled color images, 5,000 for training and 8,000 for testing. The image size is 96×96, the number of categories is 10, and each category contains 1300 i...
Embodiment 3
[0100] This embodiment provides an unsupervised deep representation learning method based on image translation, such as Figure 4 shown, including:
[0101] S11. Perform random translation transformation on the image and generate auxiliary labels;
[0102] S12. Applying a mask to the image after translation transformation;
[0103] S13. Predict the actual auxiliary label of the image after applying the mask, and learn the depth representation of the image;
[0104] S14. Updating the parameters of the deep neural network based on the loss function;
[0105] S15. Extracting representations of images.
[0106] Further, in the step S11, random translation transformation is performed on the image, and the translation transformation image is expressed as:
[0107] Among them, given an image dataset containing N samples x per image i They are all represented by a C×W×H matrix, C, W, and H are the number of image channels, width and height respectively; use Indicates the im...
PUM
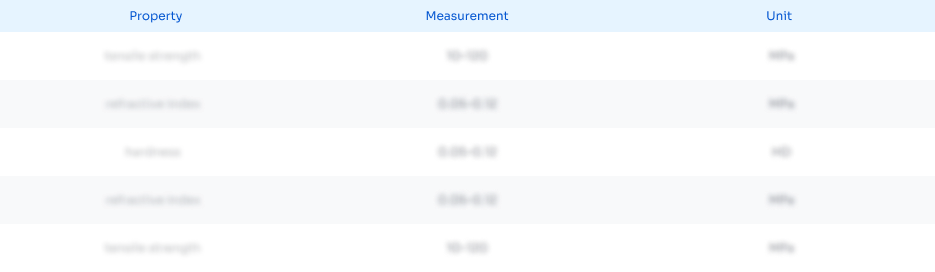
Abstract
Description
Claims
Application Information
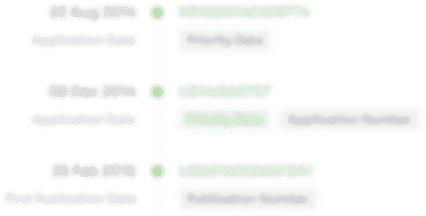
- R&D Engineer
- R&D Manager
- IP Professional
- Industry Leading Data Capabilities
- Powerful AI technology
- Patent DNA Extraction
Browse by: Latest US Patents, China's latest patents, Technical Efficacy Thesaurus, Application Domain, Technology Topic.
© 2024 PatSnap. All rights reserved.Legal|Privacy policy|Modern Slavery Act Transparency Statement|Sitemap